INTRODUCTION
Multiple stressors to coastal ecosystems associated with global change include warming waters, rising sea level, reduced oxygen, reduced pH, changing productivity, and sea ice loss (Gruber, 2011; Doney et al., 2012; Mathis et al., 2014), and these are in addition to changes in water quality associated with upland land use, nutrient pollution, and coastal infrastructure. Stressors, their interactions (e.g., Bopp et al., 2013), and the scales at which they affect ecosystems are modulated by different levels of biological organization—either through physiological shifts or changes in species assemblages. These changes in the abundance of different forms of life, or biodiversity, can be measured at several levels of complexity, from genes to populations, communities, and ecosystems.
Biodiversity is a key indicator of ecosystem health; changes in assemblages result in changing resilience, productivity, and interactions among species that can positively or negatively affect the ecosystem services that sustain human economies and social well-being (e.g., Worm et al., 2006; Duffy et al., 2013; Miloslavich et al., 2018). Satellite remote sensing is an important component of marine and terrestrial biodiversity studies (Muller-Karger et al., 2018a; Skidmore et al., 2021). It is a collection of methods that provides repeat synoptic coverage of ecosystems at multiple scales and with linkages between species-level information, such as distribution and richness, and between regional and global information, including primary productivity, nutrient cycling, and climate change (Turner et al., 2003, 2015). For oceanic habitats, where advection, mixing, and other local heat and material exchange results in shifts in the extents of habitats at subseasonal to interannual timescales and from submesoscale (hundreds of meters) to basinwide (thousands of kilometers) spatial scales, synoptic time series provide oceanographic context to ecological observation programs (e.g., Hardman-Mountford et al., 2008; Kavanaugh et al., 2016). With recent advances in spectral, spatial, and temporal resolutions and radiometric quality (e.g., Werdell et al., 2018; Muller-Karger et al., 2018a; Li et al., 2019), the scientific and conservation communities can now better measure and model community and ecosystem responses to environmental variability, including climate change.
One challenge is to establish a substantive biodiversity information base by which we can gauge changes in marine ecosystems (Duffy et al., 2013; Muller-Karger et al., 2014). In 2014, the National Aeronautics and Space Administration (NASA), the National Oceanic and Atmospheric Administration (NOAA), the National Science Foundation (NSF), and the Bureau of Ocean and Energy Management (BOEM) pooled resources under the National Ocean Partnership Program (NOPP) to initiate a pilot Marine Biodiversity Observation Network (MBON). The goal was to establish a scalable and transferable observational model for detecting biodiversity and marine habitat variability with direct application to resource management and decision-making (Figure 1). MBON nodes have been established in US coastal waters that represent polar, temperate, and subtropical ecosystems. Four nodes were established in 2014 in the Chukchi Sea, in central and southern California waters, and in the southeast Gulf of Mexico off Florida. Additional nodes were initiated in the Pacific Northwest and the Gulf of Maine in 2019. Practices for these nodes are designed to integrate existing or new data relevant to their regions and ecosystems and to share methods to establish best practices, including standardizing data and information synthesis approaches (Benson et al., 2021, in this issue) and collection of a wide array of remotely sensed biological and physical variables that contribute to understanding broad biogeographic trends across coastal systems.
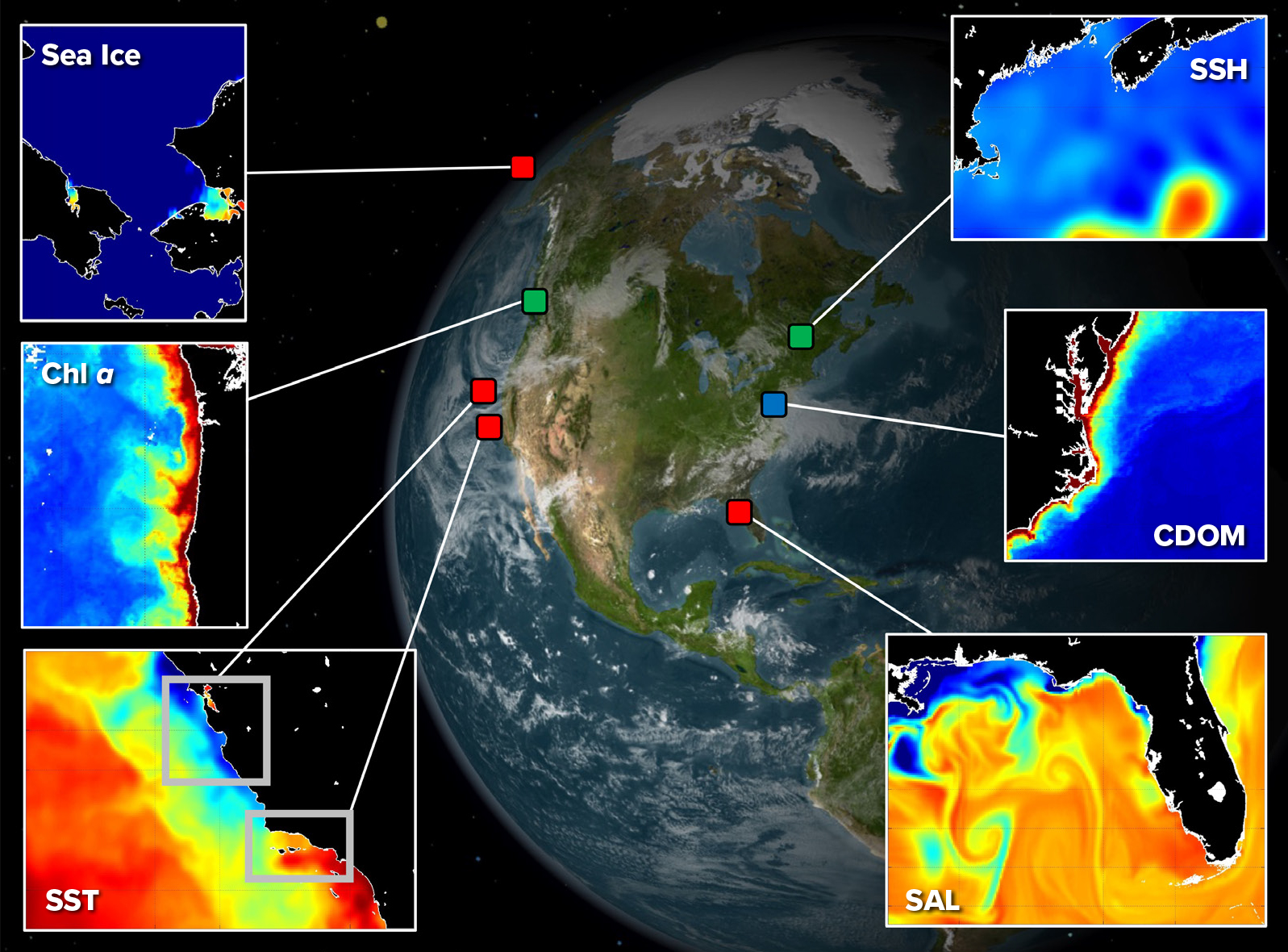
Figure 1. Satellite remote sensing and the Marine Biological Observation Network. Red squares denote the original demonstration nodes in waters of the Arctic, Central California, Southern California Bight, and Florida Keys. Green squares indicate nodes added in 2019 in the Northern California Current/Pacific Northwest and the Gulf of Maine. The blue square locates an ongoing partnership site with the Smithsonian Institution. Each node represents a unique coastal environment with variability in biophysical dynamics where in situ observations complement remote sensing. The figure highlights biological and physical variables that are all available with current remote sensing, including sea ice, sea surface temperature (SST), sea surface height (SSH), salinity (SAL), and chlorophyll a (Chl-a), along with chromophoric dissolved organic matter (CDOM) from ocean color (see section on Remote Sensing and Biodiversity: Challenges and Current Capacity). > High res figure
|
Here, we highlight where MBON research is currently addressing knowledge gaps and methodological advances that allow inferences to be drawn at multiple levels of ecological organization using remote sensing. Focusing primarily on ocean color measurements, we first describe the current suite of sensors used and the ongoing challenges with observing ocean color in complex coastal zones. We then highlight four paths where MBON research is addressing these challenges to observe biodiversity in and across optically and ecologically unique coastal environments to obtain information on plankton community composition, foundation species, dynamic pelagic habitats, and regional-scale species distributions. We then discuss recommendations that include preparing for new sensors, combining sensors for increased coverage in space and time, integrating higher taxonomic detail across multiple trophic levels, and contributing to regional to global indicators that link in-water patterns to broad-scale marine ecosystem management.
Remote Sensing and Biodiversity: Challenges and Current Capacity
Satellite remote sensing, in particular ocean color, can contribute to monitoring biological patterns and processes by providing information on biomass or dominant taxa of lower trophic levels. Ocean color instruments measure ultraviolet, visible, and near infrared light at the top of Earth’s atmosphere, and atmospheric correction algorithms are applied to obtain remote-sensing reflectances (Rrs), a measure of the color of Earth’s surface. Rrs are then related to biogeochemical quantities of interest using statistics or descriptive models. In marine environments, common biogeochemical quantities include chlorophyll a (Chl-a), the concentration of particles (inorganic and organic, including phytoplankton), dissolved colored molecules, and seawater. All these “constituents” alter light through absorption and/or scattering across different wavelengths. The physical, biological, geological, and chemical compositions of coastal and marine habitats are complex, and different processes and materials affect electromagnetic radiation differently—so measuring the complexity of these habitats requires sensors with different spatial, temporal, and spectral resolutions. Thus, a multi-sensor approach is often required for ecological studies to contend with the hydrodynamic and bio-optical complexity of coastal zones.
Currently, various remote-sensing platforms provide broad capacity for observing biophysical phenomena and patterns of interest that affect biodiversity across a hierarchy of space and time (Figure 2). Most operational products are derived from multi-spectral and passive sensors that measure changes in reflected sunlight. At the smallest spatial scales (centimeters to meters), suborbital sensors, including those mounted on unoccupied aerial systems, have been used to observe benthic habitat characteristics such as the diversity of corals and algae (including patches) present (Collin et al., 2018; Johnston, 2019; Bell et al., 2020b; Monteiro et al., 2021). While the nanosatellite constellations, for example, CubeSats, can provide high spatial (4 m) and high temporal resolution information to monitor terrestrial systems (e.g., Aragon et al., 2021), their spectral resolutions and radiometric qualities currently limit their application to marine ecosystems. However, at scales of tens of meters, Landsat has provided time series of multispectral data since the 1970s at 30 m resolution and 16-day repeat. This allows assessment of slower growing, patch-forming organisms, including large coral reefs, kelp forests, seagrasses, and mangroves, particularly with Landsat 8’s improved ocean color sensitivity (see Cavanaugh et al., 2011, 2019; Bell et al., 2018, 2020a; Hamilton et al., 2020).
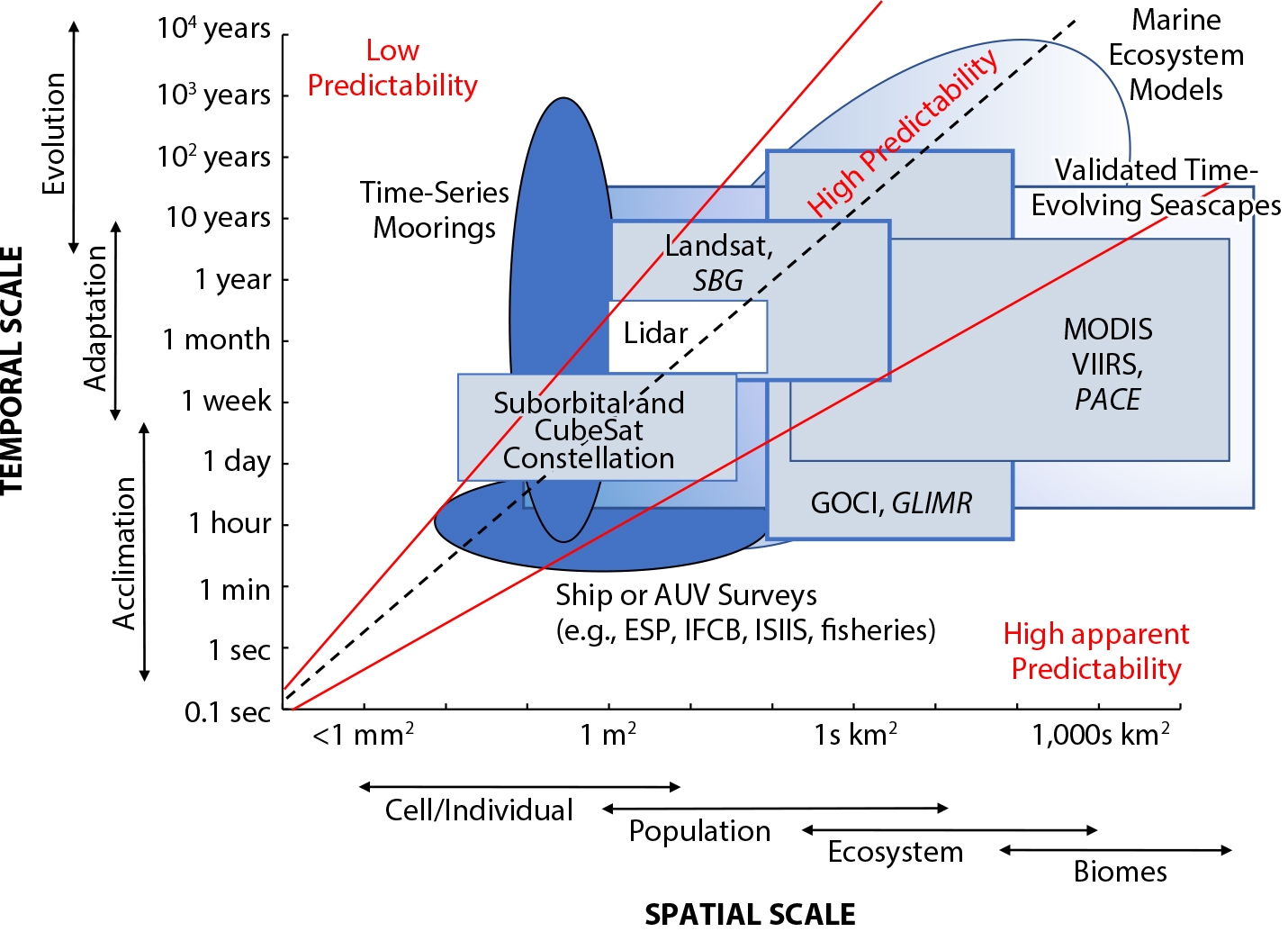
Figure 2. Stommel diagram showing the connections between satellite and in situ ocean observations and the spatial and temporal scales of biological organization and basic processes of interest. Spatial and temporal coverages highlight the current sampling capacity of the Marine Biodiversity Observation Network where in situ measurements can sample at finer spatiotemporal and taxonomic resolution. Low predictability and high apparent predictability (Wiens, 1989) denote spatial temporal scales where undersampling or naive space for time substitution may mask important ecological mechanisms. Platforms with darker shading or italicized text denote increased taxonomic resolution that can be resolved. Both current and future sensors are listed, with future sensors italicized. > High res figure
|
Over scales of hundreds of meters to kilometers, the time evolution of plankton communities can be observed over a range of temporal and spatial scales using ocean color sensors, which have increased sensitivity to account for the absorption of light by water. The Coastal Zone Color Scanner (CZCS) provided proof-of-concept with imagery collected between 1978 and 1986. Since 1997, near-daily, multispectral ocean color at ~1 km has been provided by polar orbiting satellites launched by Japan, the European Union, the United States, India, China, and several other countries. In the United States, sensors have progressed from the Sea-viewing Wide Field-of-view Sensor (SeaWiFS; 1997–2010) to the Moderate Resolution Imaging Spectroradiometer (MODIS; 2002–present), and finally to the Joint Polar Satellite System (JPSS) Visible Infrared Imager Radiometer Suite (VIIRS; 2012–present). In 2015, the European Space Agency (ESA) launched the Sentinel constellation, which provides higher spatial resolution (~300 m for Sentinel-3) and improved repeat coverage by flying sensors on two companion satellites. Repeat observations at scales of minutes to hours can be acquired for smaller regions using geostationary satellites; the South Korean Geostationary Ocean Color Imager (GOCI) provides ocean color imagery of marginal seas around the Korean peninsula approximately eight times per day.
Together, multispectral imagers have provided information on phytoplankton Chl-a (Hu et al., 2012; Siegel et al. 2013), chromophoric dissolved organic matter (Siegel et al., 2002; Werdell et al., 2013), chlorophyll fluorescence (a metric of phytoplankton physiology; Behrenfeld, et al., 2009), particle backscatter (Loisel et al., 2006; Bisson et al., 2021), and particulate organic carbon (Stramski et al., 2008). These observations from passive sensors can provide frequent and long-term measurements of surface ocean constituents to depths ranging from a few centimeters to several tens of meters, depending on the turbidity of the water and whether there is relatively high solar illumination under clear-sky conditions. Yet, they have been used to estimate primary productivity in upper ocean waters (Behrenfeld and Falkowski, 1997; Westberry et al., 2005) and the flux of organic particulate material to the bottom of the ocean (Muller-Karger et al., 2005) using simple but effective models.
Active light detection and ranging (lidar) observations complement passive ocean color remote sensing. Lidar uses light from a pulsed laser to observe the ocean, and it works during day or night, through moderate cloud and aerosol interference, and in polar darkness (Behrenfeld et al., 2017). Lidar platforms’ capacity to provide information on vertical variation in particles over the upper few tens of meters of the water column (e.g., Lu et al., 2014; Hostetler et al., 2018) allows their use as complements to passive sensor systems that can only obtain a single integrated value for surface waters. Global-scale particulate organic carbon retrievals from space-based lidar observations have been available since 2006 in measurements from the Cloud-Aerosol Lidar with Orthogonal Polarization (CALIOP) sensor (Behrenfeld et al., 2013) and more recently from IceSAT2 (Lu et al., 2020). Due to their near-nadir viewing geometry, lidar tracks have to date offered limited spatial coverage and long repeat times (16–60 days), and thus are best used in conjunction with passive sensors.
Relative to the open ocean, coastal zones have many different constituents that continuously and often rapidly change colors and turbidity conditions. Measuring this complexity requires sensors with higher spatiotemporal resolutions, radiometric qualities, and spectral resolutions (see Mouw et al., 2015, and Muller-Karger et al., 2018b, for reviews). Coastal systems are highly dynamic and contain fine-scale features (Davis et al., 2007; Moses et al., 2017). Their characteristics include tidal exchange and upwelling, riverine/freshwater inputs, interaction between water and bathymetry, and complex coastline shapes, and some locations are subject to sea ice dynamics. Coastal ecosystems are productive, with high particle and dissolved organic matter loads that attenuate light in the water, further reducing the signal of interest leaving the water (Cannizzaro and Carder, 2006; Tzortziou et al., 2007). Both benthic and plankton producers fuel coastal food webs and can drive biodiversity patterns; it is difficult to separate these signals without high spectral measurements over long time periods (Dekker et al., 2007). Nearshore suspended sediments are bright and can swamp sensitive ocean color sensors, affecting both in-water algorithms and atmospheric corrections. Dynamic and diverse airborne inputs near land, including dust, aerosols, and pollution, make atmospheric correction challenging. Water masses with different optical constituents may mix at multiple scales (e.g., Kavanaugh et al., 2014a, 2016; Palacios et al., 2012) and may be advected from their origins. Many global ocean color algorithms assume an average or constant water mass composition, which limits their direct application in complex coastal zones. MBON science seeks to address these challenges in order to meet current needs while bridging between current observational capacity and future sensors.
In marine systems, the scale of detection is affected by movement (horizontal and vertical) in addition to physical mixing processes, which effectively smear the spatial footprints and timescales to which potential mechanisms may be attributed (Kavanaugh et al., 2016); this leads to high apparent prediction (sensu Wiens, 1989) but incomplete understanding of mechanisms. Sampling the environment only at fine spatial scales typically gives little predictive capacity for large-scale processes (low predictability, Figure 2). Sampling shorter-term processes infrequently or over larger scales misses key features and characteristics of the processes being observed, aliasing signals of interest. Furthermore, variability in the vertical (e.g., subsurface foraging, diel migration, and productivity in deeper layers) is mostly invisible to satellites.
Remote-sensing tools are often used in conjunction with a network of ground observations and experiments to lend mechanistic understanding of the processes underlying patterns and to provide information from depths to which many satellites cannot see. MBON nodes are strategically linked to other programs, including the US Integrated Ocean Observing System (IOOS), the Long Term Ecological Research (LTER) network, and the National Marine Sanctuaries, along with various state and federal agencies to include additional observations, mechanistic insights, and contexts (see other articles in this issue). These groups also benefit, as they serve as the ultimate “users” of new information generated by the ability of MBON to integrate multiple information types and data streams. The exchange of data and knowledge is iterative and cooperative (e.g., Iwamoto et al., 2019), with remotely sensed and in situ data merged to meet current science and management needs.
MBON: Four Methods for Moving Forward
Remote sensing offers four methods for measuring biodiversity, three of them directly synoptic. The first derives information on phytoplankton community composition from remote-sensing reflectance spectra (see reviews in IOCCG, 2014; Bracher et al., 2017; Mouw et al., 2017). This method makes the general assumption that primary producer community composition is related to optically discernible pigments or cell structures. The second method maps and quantifies the spatial distribution and temporal dynamics of foundation species (e.g., kelp forests, coral reefs, forage species) that structure populations and communities (e.g., Angelini et al., 2011; Castorani et al., 2018; Lamy et al., 2020). The third combines biophysical information from multiple sensors to identify features (e.g., fronts, eddies) or habitat patches (e.g., seascapes) that can map the quality and geographic extents of pelagic habitats (Oliver and Irwin, 2008; Kavanaugh et al., 2014a, 2016, 2018). A fourth indirectly synoptic method utilizes relationships among remotely sensed biophysical variables and occurrences or abundances of tagged or surveyed organisms to map species distributions and biodiversity patterns (e.g., Cimino et al., 2020; Gagné et al., 2020). All four methods assume to some degree that primary producer community structure relates to the structure of higher trophic level communities and that phenological changes in primary producer communities propagate throughout food webs (Vargas et al., 2006; Chavez et al., 2011; Cermeño et al., 2013, 2014; Vallina et al., 2017; Santora et al., 2017).
Method 1. Phytoplankton Community Composition Derived from Remote-Sensing Reflectance
Phytoplankton form the base of the oceanic food web, providing food for ecologically and commercially important fisheries and fueling the exchange of carbon between the ocean and the atmosphere. Most phytoplankton are beneficial; however, some species can result in toxic or noxious blooms that can have negative immediate or cumulative effects on higher trophic levels, including humans (e.g., Stumpf, 2001; D.M. Anderson et al., 2009; C.R. Anderson et al., 2015). Thus, understanding and monitoring changes in plankton abundance and structure is a vital component of coastal ecosystem monitoring and management.
Phytoplankton species can be parsed into several classification types, based on a mixture of heuristics including size, geochemical function (e.g., silica producing, nitrogen fixing), or taxonomic groups (e.g., diatoms, dinoflagellates, cyanobacteria) that are expressed through accessory pigments or absorption spectra (IOCCG, 2014; Mouw et al., 2017; Bracher et al., 2019). While broad classifications allow detection through multi-spectral methods, there are challenges in all classification types—groupings are often overly broad and misclassified. For example, grouping cyanobacteria all together lumps genetically and ecologically distinct taxa (Scanlan and West, 2002). Classification of diatoms based on their main accessory pigment fucoxanthin is imprecise because several dinoflagellate species and some haptophytes also contain fucoxanthin (e.g., Wright et al., 2005; Dierssen et al., 2020). Furthermore, dinoflagellates themselves are not strictly phytoplankton, but they have many heterotrophic and mixotrophic representatives. Obtaining the taxonomic details of microorganisms has traditionally relied on costly and time-consuming genetic and microscopy methods, with expertise in the latter becoming increasingly rare. Thus, the longer time series that are critical for quantifying baselines and trends may only focus on imprecise classifications that characterize polyphyletic differences through marker pigments or coarse differences in spectra.
Several algorithms have been developed to relate the optical constituents (spectra, shape, pigments) of various plankton groups to multispectral remote sensing. Single bloom-forming species such as coccolithophores (Moore et al., 2012), Trichodesmium sp. (Subramanian et al., 2001; Westberry et al., 2005), or diatoms (Kavanaugh et al., 2015; Kramer et al., 2018) can sometimes be resolved, depending on local ecology. Multi-species algorithms involving dominant taxa (Alvain et al., 2008), community composition (e.g., Hirata et al., 2011; Brewin et al., 2012; Bracher et al., 2015), or phytoplankton community size distribution (e.g., Kostadinov et al., 2010, 2016; Mouw and Yoder, 2010; Bricaud et al., 2012) have been determined using spectral approaches that include both absorbance and scattering properties derived from moderate resolution remote sensing reflectances (see Bracher et al., 2019, for review). Identifying dominant phytoplankton is important for biogeochemical applications in order to identify and track harmful algal blooms and to document the distribution, extent, and phenology of the large beneficial blooms on which organisms depend. Size-class algorithms are useful because much of the ocean trophic structure depends on the size distribution of the phytoplankton (e.g., Lombard et al., 2019).
Phytoplankton community composition (PCC) algorithms vary in their degrees of spectral complexity. Two general approaches exist, abundance-based and spectral methods. Abundance-based algorithms rely on the empirical relationship of accessory pigments or size classes to the magnitude of absorption at a limited set of single wavelengths or Chl-a concentrations (Uitz et al., 2006; Hirata et al., 2011). In many regions, however, these relationships break down due to physiological variability in the ratios of accessory pigments to Chl-a (e.g., White et al., 2015), multiple co-occurring taxa containing the dominant indicator pigment and thus spectra (e.g., diatoms and some dinoflagellates), imperfect assumptions of size (e.g., diatoms can be <20 μm), or poor retrieval/high contamination of the satellite Chl-a signal. Spectral algorithms focus primarily on the variability of the absorption and scattering of particles across multiple wavelengths (Montes-Hugo et al., 2008; Kostadinov et al., 2009; Mouw et al., 2010; Catlett and Siegel, 2018). Spectral absorption algorithms focus on the spectral variation of accessory pigment absorption by different taxa (e.g., Ciotti and Bricaud, 2006; Chase et al., 2017; Catlett and Siegel, 2018) or the role of the packaging effect on phytoplankton absorption spectra (Ciotti and Bricaud, 2006; Bricaud et al., 2012). Spectral scattering algorithms include size class algorithms that exploit the relationship of particle size distribution (PSD) to the spectral slope of beam attenuation or backscattering using inversion (e.g., Loisel and Stramski, 2000; Boss and Pegau, 2001; Kostadinov et al., 2009; Lee et al., 2010; Werdell et al., 2013) or empirical relationships (Carder et al., 2004; Montes-Hugo et al., 2008; Mouw et al., 2010).
Most PCC algorithms have been tuned to large-scale global gradients, thus missing some of the optical complexity in coastal zones (Maritorena et al., 2002; Siegel et al., 2002; Werdell et al., 2013). Coastal zones tend to have strong non-algal bio-optical constituents that vary in space and time, so tuning global PCC algorithms for local coastal conditions remains an important challenge and area of active research (e.g., Kramer et al., 2018).
Ongoing MBON efforts related to satellite-derived plankton community composition focus on improving the skill of global algorithms in regional oceans, creating more quantitative test records. These efforts include repeated surveys to compare absorption spectra of blooms dominated by different taxa, including diatoms, dinoflagellates, and others, in artificial controls and natural assemblages in the Santa Barbara Chanel and the Florida Keys (Figure 3a,b; see also Catlett and Siegel, 2018; Montes et al., 2020; Catlett et al., 2021). Plankton types can be distinguished in natural assemblages by investigating spectral derivatives (Figure 3b) that reveal signals from accessory pigments of different groups. The relative contributions of algal particles, detritus, and dissolved constituents can also be compared across ecologically and optically distinct water masses (Figure 3c; see also Montes et al., 2020). These activities will all contribute to NASA’s upcoming Plankton, Aerosol, Cloud, ocean Ecosystem (PACE) and Surface Biology and Geology (SBG) missions. Both missions will include hyperspectral sensors to better discern inflections in the radiometric spectra that are caused by different taxonomic groups; these will be discussed in greater detail in a following section. Where possible, MBON activities compare and combine co-occurring records of different taxonomic and spectral resolution (Catlett and Siegel, 2018; Montes et al., 2020) to link historic ocean time series with the capabilities of future sensors.
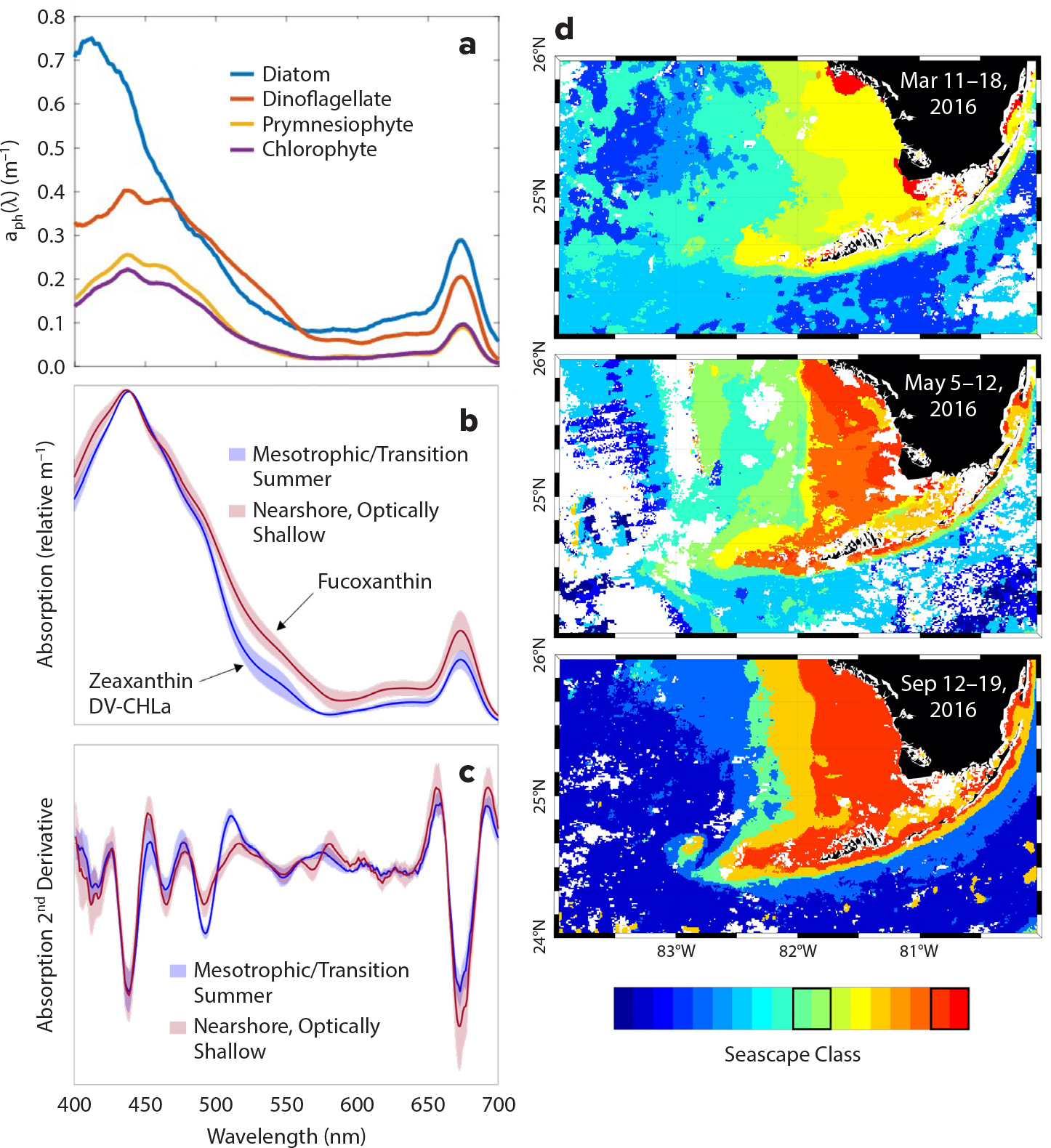
Figure 3. Development of phytoplankton community composition algorithms for temperate upwelling systems and subtropical shallow shelves. (a) Representative particulate/phytoplankton absorption spectra from blooms of four different phytoplankton groups, assessed as the highest observed concentration of each phytoplankton group’s biomarker pigment over a 12-year, approximately monthly time series from the Santa Barbara Channel, California (see Catlett and Siegel, 2018, for details). (b) Mean absorption spectra and (c) second derivatives of phytoplankton communities collected in (d) two seascape categories (highlighted with black boxes on the color bar) and seasons on the Florida shelf (see Montes et al., 2020). Second derivative analyses allow the quantification of unique, or the relative dominance of, pigment biomarkers, where fucoxanthin is indicative of nearshore diatom populations in optically shallow seascape water masses and zeaxanthin is indicative of cyanobacteria (e.g., Synechococcus sp. and Prochlorococcus sp.) > High res figure
|
Method 2. Remote Sensing of Foundation Species
Foundation species create locally stabilized environments (Lamy et al., 2020) that provide refuges (Castorani et al., 2018) or isolated patches of different, more-complex habitats that can result in increased local or regional biodiversity. In marine environments, these species include primary producers such as mangroves, kelps, and seagrasses as well as reef-forming groups such as corals and bivalves (Figure 4). Foundation species may also provide critical habitats for reproduction and population growth of ecologically and economically important fish species.
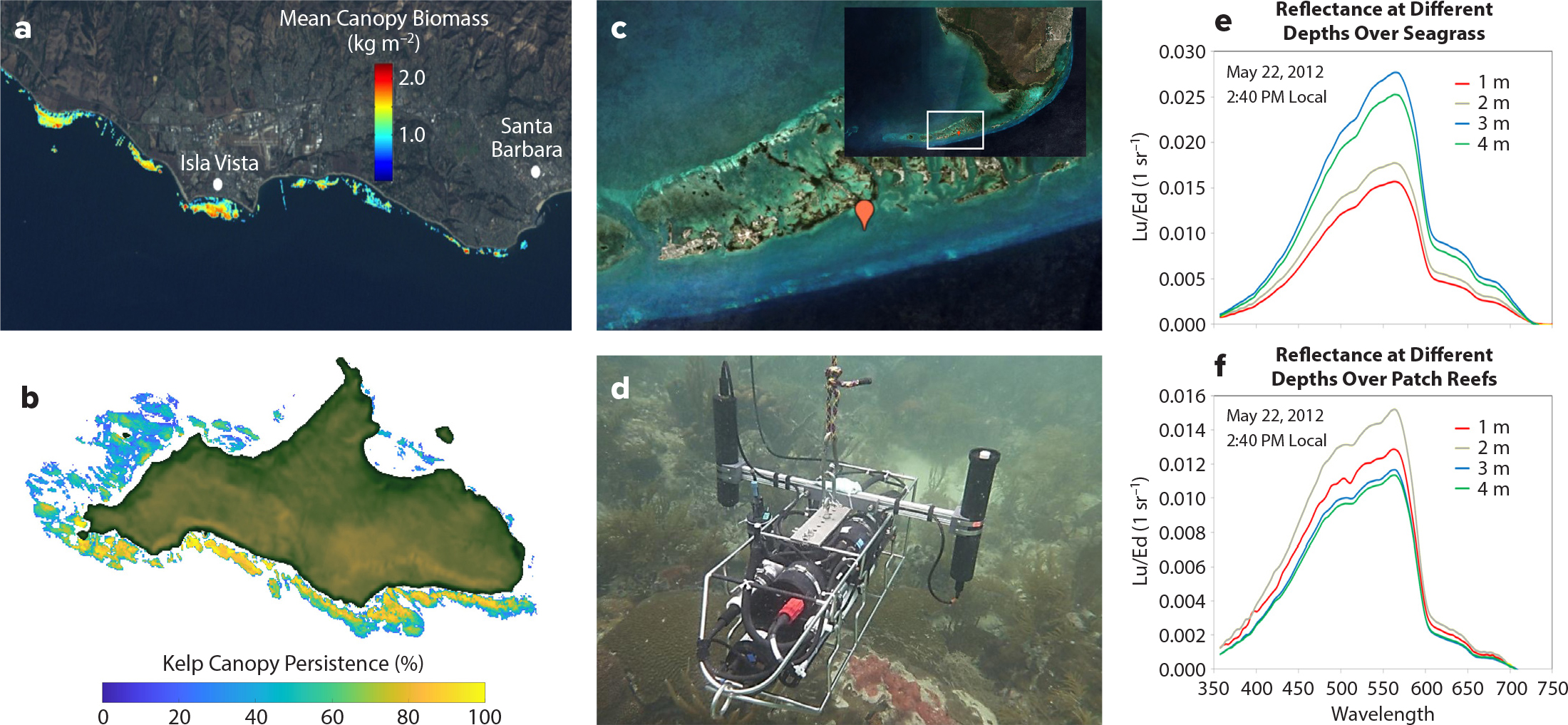
Figure 4. Development of foundation species algorithms for moderate spectral resolution, higher spatial resolution sensors. (a) Mean Macrocystis canopy biomass (Cavanaugh et al., 2011) derived from Landsat satellite sensors. Image credit NASA; SBC LTER Site (b) Kelp persistence for San Miguel Island, California, using kelp canopy data derived from Landsat sensors. Persistence is defined as the percentage of years when kelp canopy was identified in a pixel at least once during a calendar year from 1984 to 2020. (c) Sentinel-2 composite image of the Florida Keys region where MBON surveys are regularly conducted. (d) Instrument used for in situ measurements of upwelling and downwelling irradiances above a patch reef during a field campaign in May 22, 2012, near Sugarloaf Key (red marker in c). (e) Reflectances over different depths above seagrasses. (f) Reflectances over different depths above patch reefs. > High res figure
|
Field-based monitoring of foundation species can be time-consuming, costly, and spatially incomplete, and it requires access and often specialized equipment (e.g., scuba, underwater imaging) along with on-the-ground expert knowledge. Compared to environmental knowledge, remote sensing can increase coverage, be time efficient, and in some cases more accurately estimate habitat distributions (Selgrath et al., 2016). Limitations to remote sensing include incomplete horizontal or vertical coverage, challenges due to spectral unmixing, and accuracy of optical data that can be affected by depth and turbidity (Goodman et al., 2013; Hedley et al., 2016). Furthermore, extraction of taxonomic annotations from imagery can be time-consuming and computationally taxing. Nevertheless, a broad suite of remote-sensing technology that includes both active and passive methods deployed on various platforms has been used successfully (Goodman et al., 2013; Xu and Zhao, 2014; Hedley et al., 2016; Purkis, 2018).
Both suborbital and satellite platforms are common today for both active and passive remote sensing of foundation species. Airborne laser scanners are currently the best available technology for providing detailed bathymetry, geomorphology, and rugosity for water depths <50 m and surveys <100 km2 (Rohmann and Monaco, 2005; Wedding et al., 2008). Airborne hyperspectral remote sensing (also known as imaging spectroscopy) is at the technological forefront of passive optical sensing for providing the most detailed benthic habitat maps (Goodman et al., 2013; Hedley, 2018; Foo and Asner, 2019), successfully classifying benthos into bottom types such as fleshy algae, turf algae, seagrass, coral, and coral rubble (Hochberg et al., 2003). By accessing data over time, changes in benthic maps can inform changes in land use, coastal development, and reef connectivity (Mumby et al., 2004; Raitsos et al., 2017).
A good example of the characterization of habitat-forming species using remote-sensing observations is the recent use of multispectral Landsat data to assess changes in the canopy biomass distribution of giant kelp, Macrocystis (Cavanaugh et al., 2011; Figure 4a,b). MBON affiliates are building on this work to assess giant kelp population dynamics on local and regional spatial scales (Reed et al., 2011; Bell et al., 2018, 2020a) and changes in giant kelp population dynamics and higher trophic level responses to recent ocean warming events (Reed et al., 2016; Cavanaugh et al., 2019). Recent work using Landsat imagery has also extended these quantitative analyses of canopy biomass to bull kelp, Nereocystis, (Hamilton et al., 2020), and enabled the mapping of kelp populations throughout the US West Coast and Alaska over time. In the near future, the spectral capabilities available from NASA’s upcoming SBG mission (Cawse-Nicholson et al., 2021) will support remote sensing of giant kelp canopy pigment concentrations, nitrogen content, physiological condition, and rates of primary production (Bell et al., 2015, 2018, 2020b; recent work of authors Bell and Siegel). MBON research on foundation species provides important preparation for SBG and addresses challenges in integrating sensors with different radiometric qualities to assess benthic vegetation through time.
Analogous studies are occurring in subtropical waters within and adjacent to the Florida Keys, where multi-scale monitoring of seagrasses and coral types is assessed using both multi- and hyperspectral methods (Figure 4c–f). Landsat and commercial satellite imagery has been used to characterize shallow benthic habitats across south Florida waters and to assess changes in their cover extent and distribution over time due to multiple pressures that include thermal stress, water quality degradation, and disease (Palandro et al., 2003; Rohmann and Monaco, 2005). Subsurface and above-water radiometric measurements collected by MBON researchers in combination with satellite ocean color data have facilitated studies on the effect of water quality on seagrass and patch reef productivity in the Florida Keys (Barnes et al., 2014, 2015; Toro-Farmer et al., 2016; Hedley et al., 2017). Coral diversity in the Florida Keys has been linked to satellite sea surface temperature (SST), with percent cover of species of high conservation priority highest in patch reefs exposed to intermediate SST variance (~7° to 11°C; see Vega-Rodriguez et al., 2015). SST imagery is now used operationally to generate thermal stress products developed by NOAA’s Coral Reef Watch Program (Liu et al., 2018) in support of coral reef monitoring and management initiatives led by NOAA and the Florida Wildlife Commission (McCarthy et al., 2017).
Method 3. Pelagic Seascape Ecology: Tracking Dynamic Features and Habitats
Seascape ecology can be broadly defined as the reciprocal interaction of organisms with the spatiotemporal variability of their environment or habitat (Kavanaugh et al., 2014a, 2016; Pittman, 2017). Advances in observational capacity have led to improved characterization of dynamic habitat, better tracking of organisms as they move through habitats, and improved modeling of species-habitat relationships. Satellite remote sensing informs several kinds of seascape-type analyses, including identification of fronts (Kahru et al., 2012), the role of Lagrangian coherent structures (LCSs) in shaping niches and fisheries (Scales et al., 2018; Watson et al., 2018;), the use and occupancy of mesoscale eddies by organisms, many species distribution models, and the classification and identification of dynamic pelagic habitats (Oliver and Irwin, 2008; Irwin and Oliver, 2009; Kavanaugh et al., 2016, 2018). Once relationships are validated, understanding biodiversity changes through habitat alterations may allow tracking of shifting communities even when sensor limitations (e.g., spectral resolution) may not allow direct assessment of taxa.
Surface flow fields from altimetry and high-frequency (HF) radar can supply advective information to delineate features or assess underlying processes (Messié and Chavez, 2017; Matson et al., 2019; Catlett et al., 2021). For example, LCSs, areas of attraction and repulsion around frontal features, define fluid dynamical niches and contribute to regional biodiversity (d’Ovidio et al., 2004; Cotté et al., 2011; Scales et al., 2018; Watson et al., 2018). MBON researchers used a combination of ocean color and HF radar to partition the spatial from the temporal part of unique coccolithophorid blooms in the Santa Barbara Channel (Matson et al., 2019) and to attribute changes in dinoflagellate dominance to anomalous advection associated with long-term shifts in the North Pacific Gyre (Catlett et al., 2021). Mesoscale features can increase the spatial heterogeneity of water masses but also enhance dispersal (Clayton et al., 2013), structuring habitat for zooplankton and larval fishes (e.g., Govoni et al., 2010), mesopelagic communities (Della Penna and Gaube, 2020), and phytoplankton (Schulien et al., 2020), and attracting foragers or predators with diverse foraging strategies (Oliver et al., 2019). In the Gulf of Mexico, MBON researchers used time series of ocean color, sea surface temperature, and altimetry to document river and other coastal and shelf waters reaching the Florida Keys (Le Hénaff et al., 2019). Depending on seasonal extensions of the Loop Current, high Chl-a water came from the Mississippi outflow or from as far away as the Campeche Bank (Otis et al., 2019).
Satellite-derived seascapes (Figure 5a,b) have been used to track features and their variability to identify important phytoplankton assemblage habitats for fisheries (Kavanaugh et al., 2015, 2016, 2018; Montes et al., 2020). Dynamic seascapes represent unique bio-optical and physical constituents (Kavanaugh et al., 2014a,b; Montes et al., 2020) and document changes in pelagic habitat associated with seasonal, interannual, and event-scale shifts in environmental forcing (Kavanaugh et al., 2017, 2018; Santora et al., 2021, in this issue). Seascapes are classified in a dynamic and hierarchical framework using synoptic time series of SST, altimetry, salinity, sea ice concentration from microwave brightness, and several ocean color variables including Chl-a, normalized fluorescent line height, and chromophoric dissolved organic matter. Some MBON seascape case studies are focused on optimizing and comparing PCC algorithms as well as quantifying species-habitat relationships of plankton across the California Current and the Florida Keys (Montes et al., 2020), of zooplankton and seabirds in the Arctic, and of pelagic forage species in the California Current (Santora et al., 2021, in this issue; recent work of author Klajbor). In the Arctic, hotspots of benthic production and diversity identified in the shallow Chukchi and Beaufort Seas by the Distributed Biological Observatory (e.g., Grebmeier et al., 2012) are linked to different seascapes (recent work of author Kavanaugh). Most recently, seascapes characterized using vessel automatic identification system data have been utilized in fisheries conservation to track movement of fishing vessels in and out of exclusive economic zones in response to changing ocean features (Woodill et al., 2021). Seascapes are produced operationally through an MBON partnership with NOAA CoastWatch (https://coastwatch.noaa.gov/cw/satellite-data-products/multi-parameter-models/seascape-pelagic-habitat-classification.html).
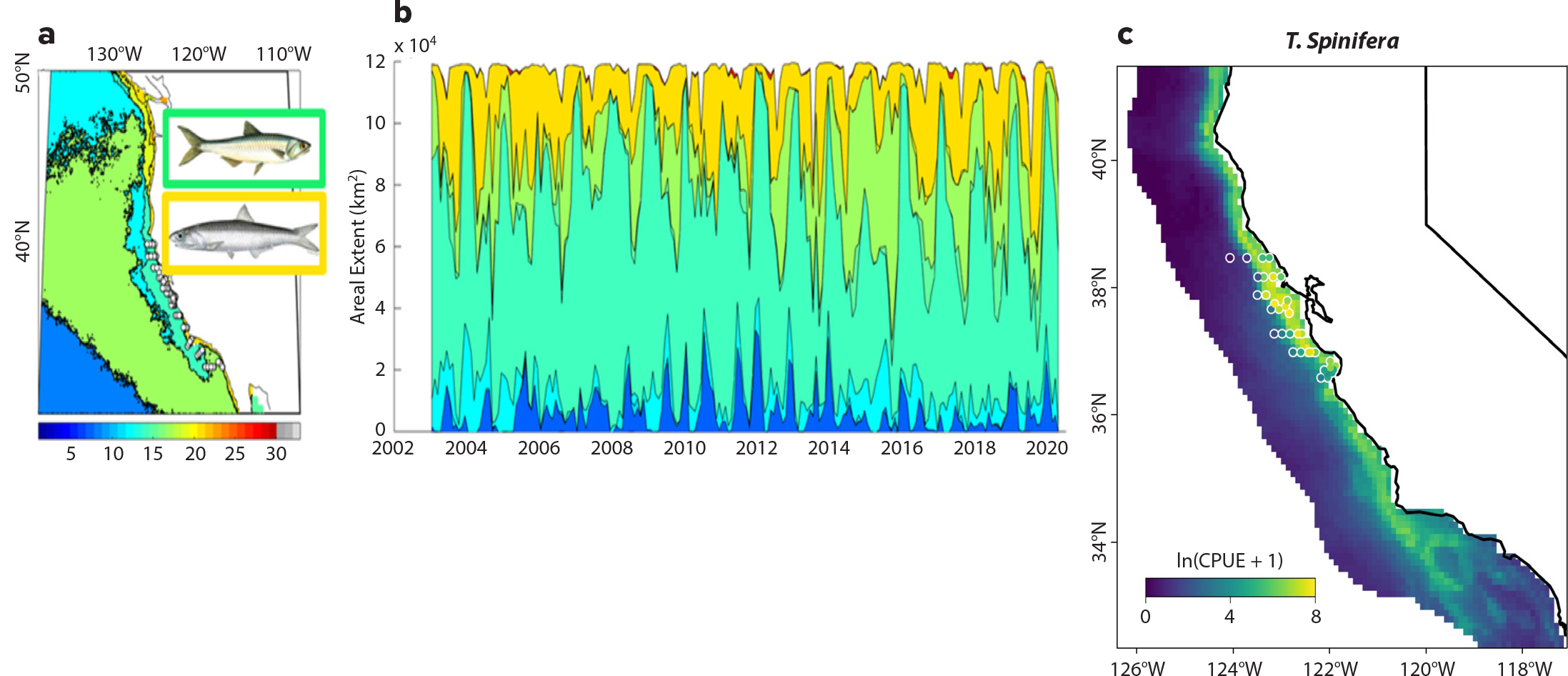
Figure 5. Multi-satellite integration to classify habitats and predict species distributions. Dominant (mode) seascape (a) and temporal variability (b) of seascape extent for the California shelf. Declines in mesotrophic (cyan) and nearshore high productivity (yellow and red) seascapes are evident during the 2014 marine heatwave. These seascapes also have different occupancies and abundances of important foragers like anchovy, sardines, juvenile rockfish, and krill (recent work of author Klajbor; Santora et al., 2021, in this issue). (c) Synoptic 2019 species distribution prediction for krill (Thysanoessa spinifera), with actual abundances of krill sampled by trawl as part of NOAA’s Rockfish Recruitment Ecosystem Assessment Surveys shown in white circles (see Cimino et al., 2020). > High res figure
|
Method 4. Species Distribution and Semi-Analytical Models
Dynamic models such as species distribution models (SDMs; Figure 5c; e.g., Cimino et al., 2020; Gagné et al., 2020) and semi-analytical models (Messié and Chavez, 2017) use environmental variables from satellite remote sensing to predict the occupancy or abundances of species ranging from plankton (e.g., Righetti et al., 2019; Elizondo et al., 2021) to higher trophic levels, including endangered species (Breece et al., 2018). Predictive models can also acquire water column information (e.g., stratification, mixed layer depth) from data-assimilative ocean models, which use satellite data (e.g., SST, altimetry) to improve model realism (Neveu et al., 2016). Species response data come in different forms (e.g., presence/absence or abundance) and originate from routine ship-based surveys, biologging, acoustics, fisheries data, or animal telemetry (e.g., Sequeira et al., 2021); all of these are types of data that many MBON partner organizations collect.
MBON affiliates have used advanced satellite-based monitoring, particularly of zooplankton and forage species. The spatial extent of krill, an important source of food for many marine mammals and birds in the California Current, was predicted in an SDM using depth, SST, Chl-a, and upwelling indices (including transport and sea surface height; Cimino et al., 2020). Satellite data-assimilative models have also documented habitat compression of forage species and calculated whale entanglement risk associated with recent marine heatwaves in the Northeast Pacific (Santora et al., 2020). Finally, semi-analytical models that retain some biogeochemical or ecological mechanisms, forced by remote-sensing products, have reproduced the spatiotemporal evolution of key plankton species such as copepods and krill (Messié and Chavez, 2017; Santora et al., 2021, in this issue) and also nitrogen fixers (Messié et al., 2020). This “growth-advection” model works by mapping the evolution of plankton communities along satellite-derived current trajectories from a known nutrient supply process (e.g., coastal upwelling along the US West Coast). Together, these models represent a complementary range of statistical (e.g., SDMs) to partially mechanistic (e.g., growth-advection) uses of remote-sensing data; a powerful approach lies in considering both together and using their agreement or disagreement as a measure of uncertainty. Furthermore, these models can also be turned into real-time or predictive decision support tools, given sustained availability of remotely sensed products (Howell et al., 2015; Hazen et al., 2018; Welch et al., 2019; Santora et al., 2021, in this issue).
MBON Next Steps: Bridging the Next Generation Satellites, Technological Advances, and Marine Ecosystem Management
MBON researchers have advanced remote-sensing capabilities in a broad spectrum of applications that can assist in understanding and monitoring patterns across several trophic levels, demonstrating that MBON can serve as a bridge between remote-sensing science, ecology, and conservation. The next steps discussed below describe four ways in which the marine biodiversity science and management communities can prepare for future science capabilities that increase spatial, temporal, and taxonomic resolution and contribute to a suite of biodiversity-relevant indicators for marine ecosystem management.
Prepare for Hyperspectral Sensors
NASA’s PACE and SBG, due to be launched in the next two years, will resolve many of the limitations discussed above and advance our capacity to view biodiversity and ocean health from space. PACE sensors include the Ocean Color Instrument, a hyperspectral radiometer that will allow scientists to better discern the minute inflections in the radiometric spectra that are caused by unique taxonomic groups. PACE will also include two polarimeters whose spatial coverage, accuracy, and spectral and angular sampling will measure diverse atmospheric constituents and improve atmospheric correction in complex coastal regions. SBG, based on the Hyperspectral Infrared Imager (HysPIRI), will complement PACE observations by collecting visible through shortwave infrared (VSWIR) and thermal infrared (TIR) spectroscopic data over shelf and coastal waters with spatial resolution (tens of meter pixels) similar to that of Landsat, revisit times of days, and temporal resolutions to weeks (Schneider et al., 2019; Cawse-Nicholson et al., 2021). As with PACE, SBG will enable improved atmospheric correction in nearshore waters and thus better-resolved PCC detection and water quality properties. Hyperspectral bands will provide the necessary information for simultaneous determinations of foundation species abundance and physiology, as well as improved benthic classifications and water quality assessments. Habitat characterization and mapping will be achieved by merging SBG records with suborbital spectroscopic measurements, such as continuous bathymetric estimates of coral reef environments. Finally, while earlier in its planning stages, the Geosynchronous Littoral Imaging and Monitoring Radiometer (GLIMR) is designed as a geostationary mission that will provide hyperspectral imagery of the Gulf of Mexico and adjacent regions. Planned to launch in 2026–2027, GLIMR will provide 10–15 observations per day to better monitor the temporal dynamics of oil spills and plankton blooms.
Recent reviews and workshop proceedings have highlighted the need for coordinated hyperspectral in situ measurements and more complex modeling with more relevant units (e.g., IOCCG, 2020) to develop algorithms that discern plankton groups and submerged or emergent vegetation (Bracher et al., 2019; Lombard et al., 2019; Dierssen et al., 2020). While some level of empirical prediction may always be necessary, particularly regionally, PCC algorithm development should emphasize bio-optical models that include aspects of radiative transfer theory (Dierssen et al., 2020), particularly if we can account for the confounding effects of unique optical water masses (e.g., Palacios et al., 2012; Kavanaugh et al., 2016). In addition, bio-optical studies can focus on the role of physiological acclimation versus community structure in driving absorption variations, on the role of heterotrophic and detrital particles in driving scattering, and on how that variability can be exploited to understand trophic processes.
In addition to in-water hyperspectral sensors and suborbital sensors on UAVs, many commercial small to medium satellite operations bridge the gap by providing short-term data sets to researchers for determining the feasibility and sensitivity of regional algorithms. While spectral and geolocation challenges affected the marine application utility of the Hyperion sensor (which flew on EO-1 from 2000 to 2017), smaller-scale, marine-focused missions have been supported by airborne sensors (e.g., the Airborne Visible Infrared Imaging Spectrometer, AVIRIS) and sensors onboard the International Space Station, including the Hyperspectral Imager of Coastal Oceans (HICO; 2009–2014) and the DLR Earth Sensing Spectrometer (DESIS, 2018–present). Together, their data archives provide a means for validating regional algorithms across a range of conditions to facilitate science- and management-ready products upon PACE and SBG launch.
Incorporate Technologies and Theory to Improve Synoptic Coverage of Multiple Trophic Levels
Technological advancements in recent years have enabled in situ, automated, continuous monitoring of biodiversity in marine environments (e.g., D.M. Anderson et al., 2012; Muller-Karger et al., 2018a,b). Increasingly, instrumentation is being deployed on mobile platforms to map the distribution and abundance of species in the ocean, some with the capability of real-time data transmission (D.M. Anderson et al., 2012). MBON researchers are currently using ship-based and autonomous instrumentation (e.g., Environmental Sample Processors, ESP; Figure 2) to collect environmental DNA (eDNA; Djurhuus et al., 2018, 2020; Chavez et al., 2021, in this issue), enumerate and identify phytoplankton and microzooplankton through imaging flow cytometry (Sosik and Olson, 2007), and survey zooplankton and ichthyoplankton with an in situ ichthyoplankton imager and sampler (e.g., Cowen and Guigand, 2008) at high frequency over large spatial scales.
Where possible, technologically advanced observations should be collected coincident with traditional methods, including microscopy, net tows, and pigment extraction (Dierssen et al., 2020), to facilitate retrospective analyses that can be vicariously validated with historic ocean color patterns. Currently, MBON scientists are conducting methodological comparisons within seascapes to determine variability of rarefaction and community composition across eDNA, high-performance liquid chromatography, and imaging-based assessments. Cross validation can also include smart, simple technology such as smart phone microscopy and spectrometry (e.g., Leeuw and Boss, 2018) that is accessible to various end users including management agencies, industry, and community scientists.
While PACE and SBG will provide unprecedented coverage of phytoplankton and larger zooplankton, forage fish are too large to be sensed with passive optical measurements and too small to be tagged. Recent advances using space-based lidar show promise for resolving course-scale differences in the timing and biomass of vertical migration (e.g., Behrenfeld et al., 2019), although variation in water mass trophic structure and/or size structure are not resolved well (Dionisi et al., 2020). Lidar algorithms cannot yet account for differences in taxa, although size distributions may be resolved by exploiting the polarimetry of multi-wavelength, ship-based lidar (Schulien et al., 2020). As a first step, MBON research will merge traditional spectral-based bio-optics, multi-trophic level imaging (e.g., Briseño-Avena et al., 2020; Schmid et al., 2020), and ecological theory to exploit signals contained within multitrophic level size distributions (e.g., Lombard et al., 2019). MBON research is also merging animal telemetry and tenets of movement ecology into a seascape ecology framework (e.g., Breece et al., 2016) to better understand behaviors that can depend on water quality and forage patchiness (Sequiera et al., 2018).
Extend Spatiotemporal Resolution with Multi-Satellite and In Situ Platform Integration
For coastal zones, the integration of open ocean satellites (both geostationary and polar orbiting) that currently have moderate spectral resolution (multispectral), moderate spatial resolution (>300 m), and high repeat (~daily or more frequent) with higher-resolution satellite (e.g., Landsat 8 30 m, 16-day repeat; pointing sensors on the International Space Station) and suborbital observations (e.g., unoccupied aerial systems, UASs) will be important for monitoring changes, particularly for interactions between pelagic processes and foundation species. Increased capacity to use UASs in coastal zones will be beneficial, along with lightweight multi-and hyperspectral sensors that can adequately detect subtle shifts in phytoplankton with improved radiometric and spectral sensitivity. Additional insight and spatial coverage can occur with a diverse suite of AUVs, including gliders, floats, and sail drones.
Over the past decade, the United States has invested in several in- and above-water observatories that provide critical and complementary observations to the MBON. On the West Coast, the Ocean Observatories Initiative (OOI) Endurance Array collects multi- and hyperspectral measurements of optical properties across Oregon and Washington shelves and has been utilized to assess regional and temporal patterns of phytoplankton biomass (Freitas et al., 2018). NOAA’s HF radar array measures water currents and waves several hundred kilometers offshore. Information on waves and water quality can inform circulation processes that affect navigation, spill responses, and general water quality, and they can also inform feature-based ecological analyses such as the accumulation of larvae along eddies, formation and evolution of local plankton blooms, and the persistence of fronts. Finally, MBON nodes each collaborate with an IOOS Regional Association so that data is exchanged between scientists and stakeholders.
Advances in modeling and integration of both predictive and mechanistic models can assist in the integration of information across sensors (including satellites), platforms, and models (Capotondi et al., 2019). Once feature space has been identified (Rose et al., 2007), model intercomparison can assist with uncertainty assessment, better parameterization of optical models, extension of properties through depth, and stronger linkages between observable patterns and mechanism. The complexity of regional and global models is expanding, and now includes parameters that are analogous to remote sensing (particularly passive remote sensing; e.g., IOCCG, 2020), as a step toward understanding the limitations of technology and algorithms in capturing future change signatures.
Contribute to Regional and Global Biodiversity Indicators
MBON nodes are partnered with National Marine Sanctuaries in the Florida Keys, Monterey Bay, and the Channel Islands, and with the new nodes in the Olympic Coast and Stellwagen Banks National Marine Sanctuaries. Sanctuaries regularly use satellite-based measurements (e.g., SST, altimetry, scatterometry) to inform Condition Reports and develop ecosystem health indicators that correspond to changes in ocean and climate (e.g., Pirhalla et al., 2009). Derived products such as SST anomalies and Bleaching Alert Areas also warn sanctuary staff regarding habitats at risk (Basta et al., 2015).
The NOAA Fisheries Integrated Ecosystem Assessment (Levin et al., 2009) includes research and synthesis to support ecosystem-based management of resources, activities, and services. While remote-sensing data are regularly utilized to identify physical changes in ecosystems, ecological indicators rely on cruise-based surveys (e.g., Fisher et al., 2015; Santora et al., 2017). For the West Coast, there are no indicators that directly connect bottom-up processes, such as currents, upwelling, nutrient loading, and temperature, to key food web groups such as zooplankton, larval fish, filter feeders, or higher trophic levels (Harvey et al., 2017). Given the relationship of phytoplankton community structure to food quality for higher trophic levels (e.g., Miller et al., 2018), the importance of monitoring for harmful algal blooms, and the increase in capacity to resolve taxonomic groups with PACE, development of satellite-based lower trophic level indicators will be important for the next phase of MBON research.
Satellite remote sensing is critical to monitoring of essential ocean and biodiversity variables, to management, and to policy-ready collections that adhere to common standards and dissemination (Benson et al., 2021, in this issue; Estes et al., 2021). The Global Ocean Observing System created the system of Essential Ocean Variables (EOVs), several of which can be observed synoptically from space. Relevant to MBON, biodiversity, and ocean health, satellite EOVs include sea surface temperature (microwave and near-infrared radiometry measurements), sea surface height and currents (altimetry), roughness (scatterometry), salinity (scatterometry and microwave radiometry), sea ice (microwave radiometry), and a broad category of ocean color (visible radiometry) (Figure 1). The diversity and biomass of phytoplankton, and foundation groups such as seagrass, mangroves, macroalgae, and corals, are also each listed as an EOV and are attainable using satellite or suborbital remote sensing.
Essential Biodiversity Variables (EBVs; Pereira et al., 2013) are indicators that can build from EOVs (e.g., Muller-Karger et al., 2018a,b) to provide information on several dimensions of biodiversity, including genetic composition, species populations, species traits, community composition, ecosystem functioning, and ecosystem structure (Schmeller et al., 2017). While MBON nodes currently measure biodiversity across all EBV dimensions, some are more directly supported by satellite remote sensing. For example, phytoplankton size is an important species trait that reflects nutrient uptake efficiencies, trophic transfer, and export of carbon (Irwin and Finkel, 2018; Lombard et al., 2019) that is measured both in situ and remotely (e.g., Kostadinov et al., 2009, 2016). Productivity and disturbance regimes are indicators of ecosystem functioning; duration, intensity, and spatial extent of anomalous events like severe storms or marine heatwaves and their effects on biogeochemistry or fisheries can all be tracked using satellite remote sensing (e.g., Santora et al., 2021, in this issue). Ecosystem structure includes habitat structure (including vertical habitat), ecosystem extent and fragmentation, and ecosystem composition by functional type. Here, MBON researchers have developed EBVs, including kelp cover (Cavanaugh et al., 2011) and dynamic seascapes (e.g., Kavanaugh et al., 2016, 2018), and thus contribute to the ability to link observations of ocean biodiversity to a global framework of marine ecosystem management and policy.
SUMMARY
Satellite remote sensing provides synoptic time series to monitor changing ocean conditions over space and time. Within the US MBON network, multi-platform satellite remote sensing is being used at local to global scales to define plankton groups; to identify the extent, composition, and functioning of foundation species as part of species distribution models; and for the classification of dynamic features and seascape habitats. Ongoing and future work will integrate high taxonomic resolution sensors and hyperspectral optics to better resolve the rich multitrophic level diversity of coastal ecosystems. As part of an integrated network, satellite remote sensing provides oceanographic context, a means to scale in situ measurements of species to communities and to broader spatial scales, and a critical tool for understanding the response of marine biodiversity to its ever-changing environment.
Acknowledgments
The authors would like to acknowledge the support of the Marine Biodiversity Observation Network (MBON), through National Aeronautics and Space Administration (NASA) awards NNX14AP62A, 80NSSC20K0017MK, NNX14AR62AFMK, 80NSSC20M0001, and 80NSSC20M008; and National Oceanic and Atmospheric Administration (NOAA) Integrated Ocean Observing System grant NA19NOS0120199. In addition, the work was supported by the Group on Earth Observations NASA awards 80NSSC18K0318 to EM and 80NSSC18K0412 to MK. FMK acknowledges the US National Science Foundation (NSF) grant 2500-1710-00 to the OceanObs Research Coordination Network, and the Gulf of Mexico Coastal Ocean Observing System NOAA Cooperative Agreement NA16NOS0120018. MM and JS were also supported by the NASA Life in Moving Oceans award 80NSSC17K0574. DS, TB, and DC acknowledge Plumes and Blumes NASA award 80NSSC18K0735, the Bureau of Ocean and Energy Management Ecosystem Studies program award MC15AC00006, NASA PACE Science Team award 80NSSC20M0226, and NSF Santa Barbara Coastal Long Term Ecological Research site award OCE-1831937.