Introduction
During the last 50 years, the world has made considerable progress in reducing the numbers and sizes of oil spills through prevention. Despite these advances, oil spills still occur, and it is critical to prepare for these events through planning and exercises that can apply the best technology and science when actual events happen. Operational decisions during deployments of spill resources are designed to expedite cleanup and minimize the overall impacts of oil spills. It is a complex process, where response decisions must consider trade-offs between a multitude of competing interests such as maritime commerce, seafood safety, human health, tourism, recreational access, habitat loss, food web disruptions, endangered species protection, and critical energy and defense infrastructure. Political interests, prescribed uses of funding, and logistic constraints can also influence what response measures are used, when and where they are deployed, and how their effectiveness can be evaluated. While planning and response tactics have evolved over time, determining what may be most at risk is often confounded by sparse background data, modeling limitations, scalability, and research gaps.
As noted in other articles in this issue, petroleum fossil fuel oils are a very complex admixture of a thousand or more chemicals that interact with physical, chemical, and geological processes on spatial scales of centimeters to hundreds of kilometers and timescales of seconds to many months. The temporal and spatial progressions of spilled oil in various states of weathering also result in varying effects on organisms, from microbes to whales, marine ecosystems, and human society (Rullkötter and Farrington, 2021; Weiman et al., 2021; Murawski et al., 2021a; and Sandifer et al., 2021, all in this issue). Over the last 10 years, the Gulf of Mexico Research Initiative (GoMRI) as well as oil spill research funded by government and private sources has helped address these issues and propelled advances in our understanding of environmental impacts and the unintended consequences of spill countermeasures, and how this understanding can be applied to developing better response, damage assessment, and restoration. The academic community and operational managers have not always interacted within the topic of oil spills due to differing objectives, but with the unprecedented amount of information resulting from GoMRI-funded research, it is imperative to bring this information into the response community’s preparedness, planning, and operational processes.
It should be noted again that this paper focuses primarily on GoMRI research and only generally refers to other oil spill research, including work that supported the US Natural Resource Damage Assessment (NRDA). The reader can find additional references and resources in academic journals related to oil spill research as well as composites of government, industry, and academic research found at the following websites:
Operational Oil Spill Modeling
Deepwater Horizon (DWH) demonstrated the strengths but also exposed some of the limitations in the deep-sea blowout oil spill modeling capability of the United States, and more specifically of the National Oceanic and Atmospheric Administration (NOAA). Since DWH, incorporation into models of deep-sea and water-column fate and effects, three-dimensional capability, faster processing, improvements to algorithms, and more user-friendly displays has provided critical updates that render the models more useful for response plans, exercises, and actual oil spills. In addition, new parameterizations and methods have been developed that can be integrated into operational models in the near future. New transportation routes and lease sites associated with changes in energy production provide additional modeling opportunities, but certain limitations and variabilities still exist in spill prediction that will need to be addressed in future research. For a more detailed summary of operational modeling, see Barker et al. (2020). The response to a significant oil spill is a large and complex endeavor, requiring many decisions that balance trade-offs between a multitude of competing interests. Each of these many decisions must be made with some understanding of how the spill unfolds and might unfold in the future, and how response actions may improve or adversely impact the environment. Predictions developed from oil spill models can help guide these decisions and are used to support planning for spill response, environmental impact analyses, response decision-making, and determining public compensation for injuries from an event through the NRDA.
This section focuses on “operational” spill modeling, which must work on short timescales (hours/days) to support operational response decisions. How oil moves (transport) and how it is transformed (fate) is governed by ocean currents, surface winds, wave climate, and water temperature. Oil spill modeling relies on predictive numerical models related to these parameters. These models use simulations to solve complex equations that describe the phenomena of interest and have the advantage that they can be employed for past (hindcast), current (nowcast), and future (forecast) applications. Oil spill transport models guide the response through forecasting the potential locations of oil. Modeling the fate (“weathering”) of oil can predict what harm the oil could cause, informing the efficacy and effects of potential response actions. Enhancing the capabilities and accuracies of spill models requires improvement of the corresponding inputs (Figure 1). It should be possible to display model results in a common operational picture (COP) along with other important environmental, safety, and response information in order to plan and prioritize the choice and deployment of response equipment and options.
Ocean circulation models provide an important basis for oil spill prediction, and several such applications were employed during DWH. Examples include the global HYbrid Coordinate Ocean Model (HYCOM; Chassignet et al., 2007), the Gulf of Mexico HYCOM (Mariano et al., 2011; Le Hénaff et al., 2012), the South Atlantic Bight-Gulf of Mexico model (Hyun and He, 2010), the Real-Time Ocean Forecast System for the North Atlantic Ocean (Mehra and Rivin, 2010), the intra-Americas-Sea Nowcast/Forecast System (Ko et al., 2008), and the University of South Florida West Florida Shelf model (Barth et al., 2008). Even though they were valuable tools for the immediate response, these models had limitations that influenced oil spill predictions. Many limitations of these models are still valid today, but recent advances have been made to overcome them, and progress continues (Fox-Kemper et al., 2019).
The sources of errors in ocean models may derive from both deterministic processes (such as tides, wind-driven events, and coastal freshwater flows) and instabilities (e.g., mesoscale eddies, submesoscale eddies, and associated fronts). For deterministic processes, the limitations in ocean model performance are controlled by model resolution, accuracy of model inputs, and ability to represent/parameterize the appropriate physical processes. Data-assimilative models improve accuracy, but are often subject to limited availability of observations, especially in subsurface/deep environments. Since DWH, most of the improvements in representations of the complex oceanic features that can drive oil movement are the direct outcome of more powerful computing platforms that allow for increased horizontal resolution. We can now perform basin-scale simulations (e.g., North Atlantic and the Gulf of Mexico) with grid spacing on the order of 1 km (submesoscale resolving; Le Hénaff and Kourafalou, 2016; Chassignet and Xu, 2017; Jacobs et al., 2019) and regional/coastal simulations with grid spacing on the order of 100 m (Capet et al., 2008). There remain deployment issues, and currently it is not practical to run such high-resolution models for every place there might be an oil spill. Rapidly addressing a future event requires having systems in place that can quickly set up a new high-resolution operational region nested in the existing larger-scale operational models. Freshwater flow presents additional challenges, as ocean models typically have represented it at only a few particular points and often at very course temporal resolution. The method employed and the parameterization of related processes can impact output on the circulation and transport in areas of river influence (Kourafalou et al., 1996; Schiller and Kourafalou, 2010; Tseng et al., 2016). Improved inputs on atmospheric and river forcings, in combination with high spatial resolution, will be necessary for accurate transport forecasts in ports and other areas that are influenced by freshwater and also likely to have spills.
Despite the new achievements in ocean models, computational capability limits models from explicitly representing processes at all the scales that control ocean circulation. As shown in detailed measurements around the DWH site by Poje et al. (2014), the influence of ocean processes on surface material dispersion increases enormously at small scales. One effect of this is enhanced dispersion over what would be predicted from larger-scale circulations. Submesoscale processes are typically much smaller in scale and have much weaker currents than mesoscale features; however, the effects on surface transport can be stronger than those coming from the mesoscale and can strongly influence oil fate by either spreading or concentrating the oil (Roth et al., 2017; Rascle et al., 2017; and Androulidakis et al. 2018).
Velocity structure in the upper meter of the water column has also been identified as particularly important in transport of spilled oil but has not been well represented in ocean models. While the ocean surface often garners the majority of attention, another critical component of accurate modeling and prediction of oil spills is deeper mixing and transport by ocean currents. Before DWH, a number of three-dimensional operational circulation models were available, but none of them could well capture the circulation at depth (MacFadyen et al., 2011). Even now, very few areas have real-time current measurements below 1,000 m, which will be critical for tracking subsurface plumes and forecasting oil movement from future deepwater releases. With higher model resolution and more powerful computing platforms, new classes of physics become resolved (Barkan et al., 2017), but high-resolution modeling requires high-resolution input data. Much of the GoMRI work showed the importance of submesoscale effects on transport and dispersion (D’Asaro et al., 2018). Targeted high-density observations allowed corrections at smaller scales so that greater forecast skill could be achieved (Carrier et al., 2016). Surface drifters can provide significant observational coverage over an area of high interest at a relatively low cost (Muscarella et al., 2015). Airborne observations could be used to provide better high-resolution data on currents for model initialization (Rodriguez et al., 2019), while satellites can provide high-resolution data with continuous global coverage (Rodriguez et al., 2018).
Prior to DWH, most operational oil spill modeling included wave transport only as part of a wind drift parameterization. This is an effective and practical approach, but as higher-resolution operational wave models come online, wave effects can be better captured (Weber, 1985). Spill models will also benefit from more closely coupled atmosphere-ocean-wave models such as the Coupled Ocean Atmosphere Prediction System (COAMPS; Allard et al., 2014). Blowout plume models were unable to answer some of the unanticipated questions that arose during DWH, including the effects of multiphase flow, dissolution of oil and gas within the plume, and droplet size distribution. Recent model development has focused on computational fluid dynamics (CFD) models of the three-dimensional multiphase plume flow field (Fabregat et al., 2015, 2016, 2017; Fraga and Stoesser, 2016; Fraga et al., 2016; and Yang et al., 2016). While these models are too computationally expensive to be deployed operationally, aspects of the physics investigated with these models can be leveraged for use in response models. Additionally, sophisticated integrated blowout plume models have been developed. NOAA has integrated the Texas A&M Oil spill Calculator (TAMOC; Dissanayake et al., 2018) with its General NOAA Operational Modeling Environment (GNOME) suite that can be run on operational timescales to predict the behavior of the plume and the properties of the oil once it reaches the surface (Gros et al., 2018).
When oil is mixed with water in a turbulent environment, either in a blowout plume or at the surface in breaking waves, it is broken down into small individual droplets. The resulting droplet size distribution has a very large influence on the fate and transport of the oil (Thrift-Viveros et al., 2015; Gros et al., 2017; Socolofsky et al., 2019). Models for droplet formation at the surface had been developed and used in oil spill models for decades (Delvigne and Sweeney, 1988), but there were not many estimates for a deepwater well release. One model (F. Chen and Yapa, 2007) did estimate droplet size but did not account for the resulting droplet size distribution after application of dispersants, either at depth or at the surface. Recent work on mechanistic models such as VDROPJ (Zhao et al., 2014) and Oildroplets (Nissanka and Yapa, 2016) improved prediction of droplet size distributions under various conditions. These models may not be suitable for real-time operational predictions but have been invaluable in better understanding droplet formation and the effects of dispersant use, including reduction of volatile organic compounds at the surface (Gros et al., 2016). For operational use, a simpler class of droplet size distribution models, fitting distribution to laboratory data, has been developed and is being deployed in current operational oil spill models (Johansen et al., 2013; Li et al., 2017).
DWH provided an opportunity to study biodegradation on a large scale, and with new tools available (genomics), it has become clear that under certain conditions, biodegradation can be a significant process at fairly short timescales. As the application of dispersants at depth could influence biodegradation and, therefore, oxygen demand (Brakstad et al., 2020), biodegradation is an important factor to include in operational modeling, and advanced biodegradation models based on droplet size are now making their way into operational models (Brakstad et al., 2015, 2017; Thrift-Viveros et al., 2015). DWH also placed a focus on the marine oil snow sedimentation and flocculent accumulation (MOSSFA) event that continued for several months after DWH was capped (Passow et al., 2012; Brooks et al., 2015, Romero et al., 2017; Daly et al., 2016; Stout et al., 2017; Stout and German, 2018; Langenhoff et al., 2019; Passow and Stout, 2020). This process had not been included in operational response models, and GoMRI research devoted to the MOSSFA process illustrated that it is very complex and difficult to model.
Models have been developed using coagulation theory to study marine particle size distributions (Jackson, 1995; Jackson and Burd, 1998), but these are not suitable for operational models. Work is ongoing to develop simplified MOSSFA models that can be incorporated into operational models. As discussed above, when modeling spill transport, the greatest limitation is accuracy and precision of the models that provide the drivers (winds, currents, waves). Therefore, spill models must use parameterizations of processes not captured by those models, such as subgrid-scale diffusion. More work needs to be done to better capture spatially variable and non-isotropic diffusion.
The GoMRI-sponsored Consortium for Advanced Research on Transport of Hydrocarbon in the Environment (CARTHE) field drifter experiments provide a rich data set that can inform the development of more sophisticated parameterizations of diffusion at the surface. For vertical mixing, improvements in near-surface diffusion will be incorporated into operational models (Nordam et al., 2019a,b). Near the surface, many transport and weathering algorithms require an estimate of the film thickness or surface area. The effect of wind on spreading has been quantified by the work of Zeinstra-Helfrich et al. (2017), and it may make its way into operational models. However, the patchiness of oil distributions is driven by submesoscale processes, such as Langmuir circulation, fronts, and small eddies. Much work has been done by GoMRI researchers on quantifying and better understanding these submesoscale circulations, but this work has not yet made it into parameterizations that can be included in operational models.
Knowledge of the fate of oil is critical for informing choices regarding response equipment and methods. New analytical techniques have greatly expanded understanding of photo-oxidation (Ward and Overton, 2020), which is thought to be important in the formation of emulsions, oil solubility, and toxicity. The implications for oil transformation on parameters important to response are not understood well enough to be included in response models, but current effort is being devoted to modeling of emulsion formation.
There will always be uncertainty in models. This uncertainty needs to be quantified and communicated to responders who rely on forecasting from those models to make decisions. One of the best ways to capture uncertainty is through ensemble modeling, which can quantify and reduce the uncertainly of forecasts (Brassington, 2017). Ensembles were used in an informal way during the DWH event (MacFadyen et al., 2011), but as more models come online, operational modeling centers are developing methods to better utilize ensembles during spill response. In the 10 years since the DWH event, the GoMRI program and other funders have supported advancing understanding of aspects of oil spill science and modeling, from individual droplets to oil slicks and biological effects of oil on wildlife and humans. This large collective focus over such an extensive set of scientific fields with multiple countries involved has set a new benchmark for knowledge in oil spill modeling and assessment. This legacy should be well used by future researchers and responders to improve oil spill modeling and response.
Improvements in Modeling Environmental Impact and Damage Assessment
As spill models improve, it is equally imperative to understand the expected impacts from the released oil in order to make informed decisions on appropriate response priorities and provide validity to the NRDA process. For example, given the extensive research on dispersants that has led to improved understanding of physical and chemical processes associated with them, the expected benefits and impacts of this countermeasure must be assessed against mechanical recovery of oil and other alternative actions such as in situ burning that also have limitations and potentially deleterious effects. The ability to predict and model ocean and coastal impacts through the nexus of laboratory research, mesocosm studies, and field measurements must be incorporated into models that will drive the environmental trade-off discussion.
As discussed, models have been refined to simulate oceanographic physical processes from the deep sea to the ocean surface where they are impacted by wind, waves, and surface ocean currents (e.g., Prasad and Hogan, 2007; Chassignet et al., 2009; Le Hénaff and Kourafalou, 2016; Weisberg et al., 2016; J. Chen et al., 2019). As study of the DWH spill progressed, it became apparent that the formation of a large undersea plume had also been important to representing oil dispersal and determining which organisms and biomes were exposed to oil (Camilli et al., 2010; Liu et al., 2011; Romero et al., 2018). However, representing undersea plume dynamics proved challenging for the physical models and required a sophisticated representation of the interplay of gas and oil, the preferential solubility of oil components, effects of dispersant on droplet size distribution, gas hydrate formation, behavior of oil and oil-degrading microbes under high pressure, oxygen consumption, and other factors.
Additional advancements were made in understanding and modeling biodegradation and chemical weathering processes, including volatilization, dissolution, and photodegradation. Processes associated with microbes also produced MOSSFA, which enhanced the sedimentation of oil. MOSSFA contributes toward the oil burden of the ocean in addition to the submerged and sunken oil that originates from the weathering process. A carefully coordinated program of field observations and laboratory experiments was critical in allowing models to accurately represent oil fate and associated injuries (Figure 2).
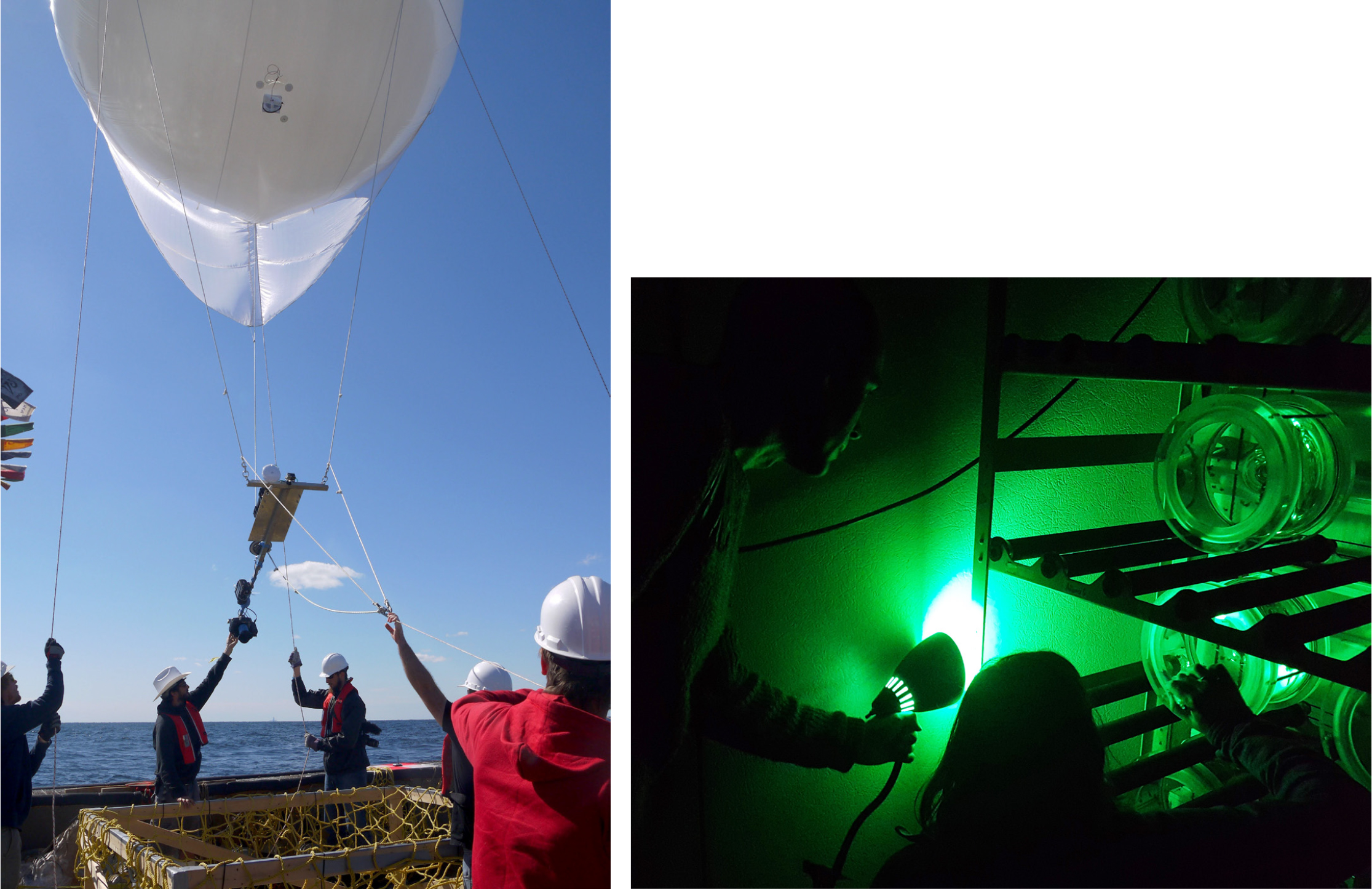
Figure 2. (right) Scientists conducted rolling table experiments to improve understanding of how marine oil snow forms and to provide input parameters for models that predict oil transport via sinking marine snow. Photo credit: Julia Sweet (left) CARTHE researchers launch the Ship Tethered Aerostat Remote Sensing System (STARSS) from M/V Masco VIII during the Lagrangian Submesoscale Experiment (LASER). STARSS was part of a large-scale oceanographic campaign designed to observe oceanic dispersion over a wide range of spatial and temporal scales and understand transport and dispersion of oil and other buoyant materials. Photo credit: Maristella Berta, CNR-ISMAR, Venice, Italy. > High res figure
|
As mentioned above, in addition to GoMRI-funded research, other extensive studies supported as part of the DWH NRDA resulted in the publication of numerous technical reports and journal articles related to oil spill modeling. Many of these are listed on the US Department of the Interior website https://www.doi.gov/deepwaterhorizon/adminrecord and in the reference section of the National Academies dispersant report (NASEM, 2020). These studies of DWH stand as examples of fruitful cooperation between empiricists and modelers. They represent considerable advancement in our ability to simulate transport and fate of oil (e.g., Reed et al., 2000; Beegle-Krause, 2001; Warner et al., 2010; Liu et al., 2011; Paris et al., 2013; Fabregat et al., 2017; French-McCay et al., 2019).
Nevertheless, of the many processes that influence the transport and fate of oil, some are yet to be integrated into larger-scale oil spill models (Spaulding, 2017). Despite impressive instances of the coupling of models by many different GoMRI authors, there remains a need to combine known processes across disciplines into a common predictive framework, for example, synthesizing ocean hydrodynamics, atmospheric dynamics, and physical, chemical, and biological process that influence oil weathering. There is also a need to further examine, for example, nearshore processes and the effects of freshwater fronts (Kourafalou and Androulidakis, 2013; Xia et al., 2020), which ultimately impact the beaching of oil in varying coastal environments. Oil beaching plays a role in a cascade of additional processes that further impact short-term mitigation and ultimately society as a whole.
In addition to the physical-biogeochemical processes that govern oil spill transport and fate, preparedness and planning for future oil spills should also consider the impacts of oil on additional knowledge domains. These additional domains include ecosystems (considers all living organisms and biotic and abiotic factors that influence their existence), socioeconomics (considers social, cultural, institutional, and economic factors that influence productivity within a community), and human health (considers both the physical and mental health effects associated with direct and perceived exposures to oil).
Some integration of physical-biogeochemical processes and biological systems has occurred through modeling efforts, for example, Atlantis, Connectivity Modeling System (CMS), Spill Impact Model Application Package (SIMAP), and Consortium for Simulation of Oil-Microbial Interactions in the Ocean (CSOMIO). These models simulate impacts in different environments, effects on organisms at different trophic levels, and the movement of organisms between different ocean regions (in some cases through passive transport by ocean currents and in others through the active movement of the organisms). For example, Atlantis (Fulton et al., 2005; Ainsworth et al., 2015, 2018) relies on coupling with hydrodynamic models for the purposes of representing the connectivity of populations and estimating oil exposure and impact. In some cases, modeling frameworks such as this allow injury assessment and also permit the exploration of different mitigation strategies. This is the case in Berenshtein et al. (2020a) and Suprenand et al. (2020), who explored possible impacts from hypothetical oil spills, and French McCay et al. (2017), who evaluated the net benefits of dispersant use. One of these models, CMS, ventures further from the physical-biogeochemical processes through biological systems and into the socioeconomics domain. This model simulates fishery capacity and evaluates the influence of fishery closures in addition to oil impacts on fishing stocks. In other examples, output from ecological models can be translated into socioeconomic impacts through coupling with input-output models (Court et al., 2019). Vulnerability indices are then defined for Gulf coastal counties, depending upon their socioeconomic conditions and the impacts of closures or changes in fishing stocks induced by the oil spill (Paris et al., 2012, 2013; Berenshtein et al., 2019, 2020a,b).
Although there has been considerable progress in integrating models across the domains of knowledge, no fully quantitative model yet exists that simulates oil spill impacts across physical, biological, socioeconomic, and human health knowledge domains. GoMRI synthesis and legacy efforts have focused on developing and exploring a framework for assessing a model that is useful for decision-making in the context of minimizing societal impacts from oil spills in the longer term. The framework is based upon a systems dynamics approach that begins by evaluating the interdependencies of the systems to be linked (Brennan et al., 2019). The causal loop diagram developed for this effort is intricate, with key processes that can be separated by domain or pairs of domains on the timescales of years, plus overall long-term (decadal) societal-level processes integrating all four domains into larger-scale loops (Figure 3; recent work of author Solo-Gabriele and colleagues).
For example, in the physical-biogeochemical process domain, the short-term operational models are utilized to simulate the hydrodynamics that influence the transport and fate of oil, and the processes that govern transport and fate are tightly integrated. Feedback between models relies on interlinked processes that impact oil transport and degradation inclusive of dispersant use and cleanup efforts aimed at mitigating shoreline impacts (represented by the large blue arrows in Figure 3). Similarly, processes influencing biological ecosystems are also tightly interlinked through interdependencies of species within various trophic levels and habitats (represented by the large green arrows in Figure 3). Ecosystems are influenced by toxins in the oil, which are impacted by physical-biogeochemical processes. Likewise, oil in the physical-biogeochemical environment is influenced by microbial degradation, which is part of the biological system processes. These two domains of knowledge, although each characterized by strong internal feedback mechanisms, thus have interdependencies that can serve to link the processes within each domain.
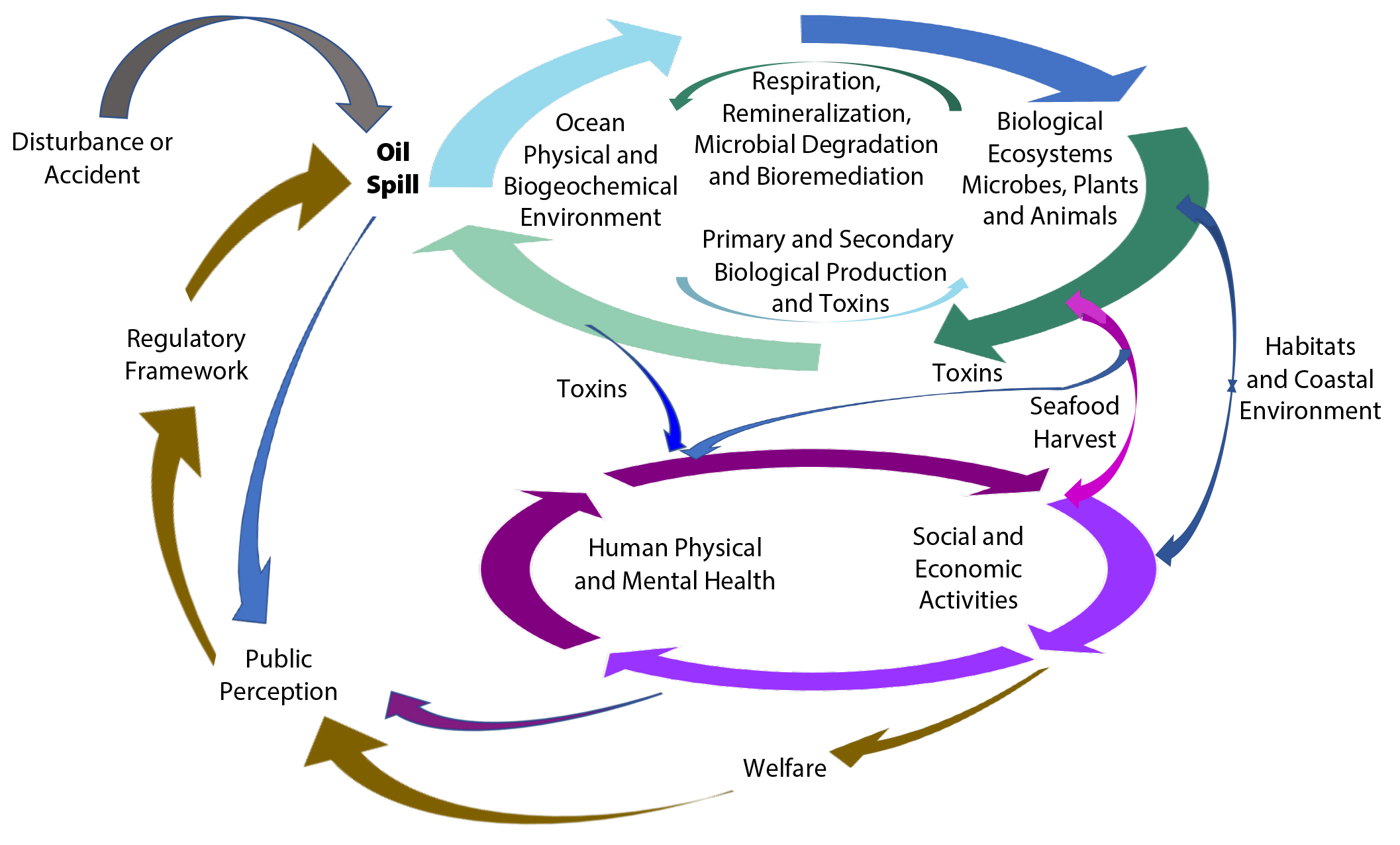
Figure 3. Framework for an integrated model for preparedness and planning of oil spill response. This framework is focused on facilitating decision-making that integrates long-term (decadal) impacts on physical-biogeochemical processes, biological systems, socioeconomics, and human health. > High res figure
|
However, the human-focused domains of socioeconomics and human health are much more intertwined. Strong feedback loops cut across these human-focused domains, as human health is defined by both physical and mental health status, with each influencing the other (McEwen and Stellar, 1993; Seeman et al., 2001; Summers et al., 2018; Koliou et al., 2018; Ferguson et al., 2020; Sandifer et al., 2020). Societal factors influence mental health through general well-being that depends heavily upon income and employment, productivity, perceptions of harm, loss of housing stability, and loss of place and valued natural resources (Abramson et al., 2015; Sandifer, et al., 2017; Guo et al., 2018). Mental health impacts affect physical health, which in turn impacts productivity, with considerable socioeconomic consequences. Feedback is thus strong between human health and socioeconomics such that processes within the lower two domains of knowledge in Figure 3 are more completely intertwined. Additionally, these human-focused processes, although known in qualitative terms, are greatly lacking in terms of quantitation. Outside of traditional risk assessment approaches for evaluating physical health impacts (Black et al., 2016) and available economic models (Court et al., 2019; Sumaila et al., 2012), there is very limited quantification of processes needed to understand relationships between each of the processes that influence socioeconomics and human health. More work is needed to understand the links among physical health, mental health, community well-being, and societal factors. The feedback loops between human health and socioeconomics are considered strong. However, processes within these knowledge domains are also influenced during oil spills by physical-biogeochemical processes and biological systems and vice versa. For example, the human-focused domains influence the physical-biogeochemical and biological systems through decisions about mitigation efforts and pressures on seafood harvesting. During an active spill, the upper tier natural processes more strongly influence the lower tier human-focused processes, where oil spills influence the exposure of humans to toxins either through direct contact with oil or indirectly through consumption of seafood. This exposure then influences socioeconomics through restrictions on the use of natural resources via declines in seafood harvest and coastal resources and through beach closures.
Ultimately, there is a longer feedback loop that operates at decadal scales. This loop, based upon perceptions of oil spill effects on community welfare, influences the regulatory framework through which the short-term operational models are mandated (brown loop in Figure 3). Consequently, outputs from the short-term operational models not only influence how rapidly society responds to protect resources that are sensitive in the short term but also influence the longer-term socioeconomics and human health domains, which in turn feed back to the regulatory framework through public perceptions of oil spill effects.
In order to consider the holistic impacts of decisions made at the short-term operational scale, models are needed that integrate physical-biogeochemical processes, biological systems, socioeconomics, and human health. Although work is needed to further understanding of all four knowledge domains, those most lacking sufficient information to create quantitative models are the human-focused domains of socioeconomics and human health (purple loops in Figure 3). The influence of these domains on the regulatory framework that influences whether or not an oil spill occurs (brown loop) also needs to be better understood in order to develop holistic decision-support models capable of assessing the effects of short-term decisions on longer-term societal impacts. Ideally, a fully developed systems dynamics model should be created to evaluate possible long-term outcomes from shorter-term decisions for immediate mitigation. Ultimately, it would be useful to link short-term operational models (as outlined above; Barker et al., 2020) to a systems dynamics model to create an integrated assessment model designed to evaluate long-term societal outcomes inclusive of socioeconomics and human health.
Choosing Response Countermeasures (Mechanical Recovery, Burning, and Dispersants)
Future integration of models can better reflect impacts and help responders choose the optimum suite of countermeasures to expedite cleanup and minimize damage. However, decisions will still need to be made based on prevailing conditions at the time of an incident, including the use of chemical dispersants as countermeasures (Quigg et al., 2021, in this issue). While questions remain, if a spill were to happen, dispersant stockpiles and equipment are available, and the expected effectiveness, detrimental effects, and unknowns must be weighed against other alternatives, as well as the potential impacts of not using dispersants. Open-ocean spills may preclude recovery by mechanical equipment or in situ burning in certain wave and weather conditions where dispersants (surface and subsea) may still be effective (Figure 4). Even if mechanical equipment is utilized, the oil recovery rates may be insufficient, and decisions will have to be made on the use of alternatives. Chemical dispersant use is predicated on the decision that overall environmental and/or worker health impacts (associated with volatile organic compounds) are reduced by dispersing oil into the offshore water column, thus reducing the impact to the nearshore and coastal areas. Surface applied chemical dispersants, such as aerial sprays, may effectively cover much larger surface areas, but their use is time critical and therefore subject to prevailing conditions and oil weathering.
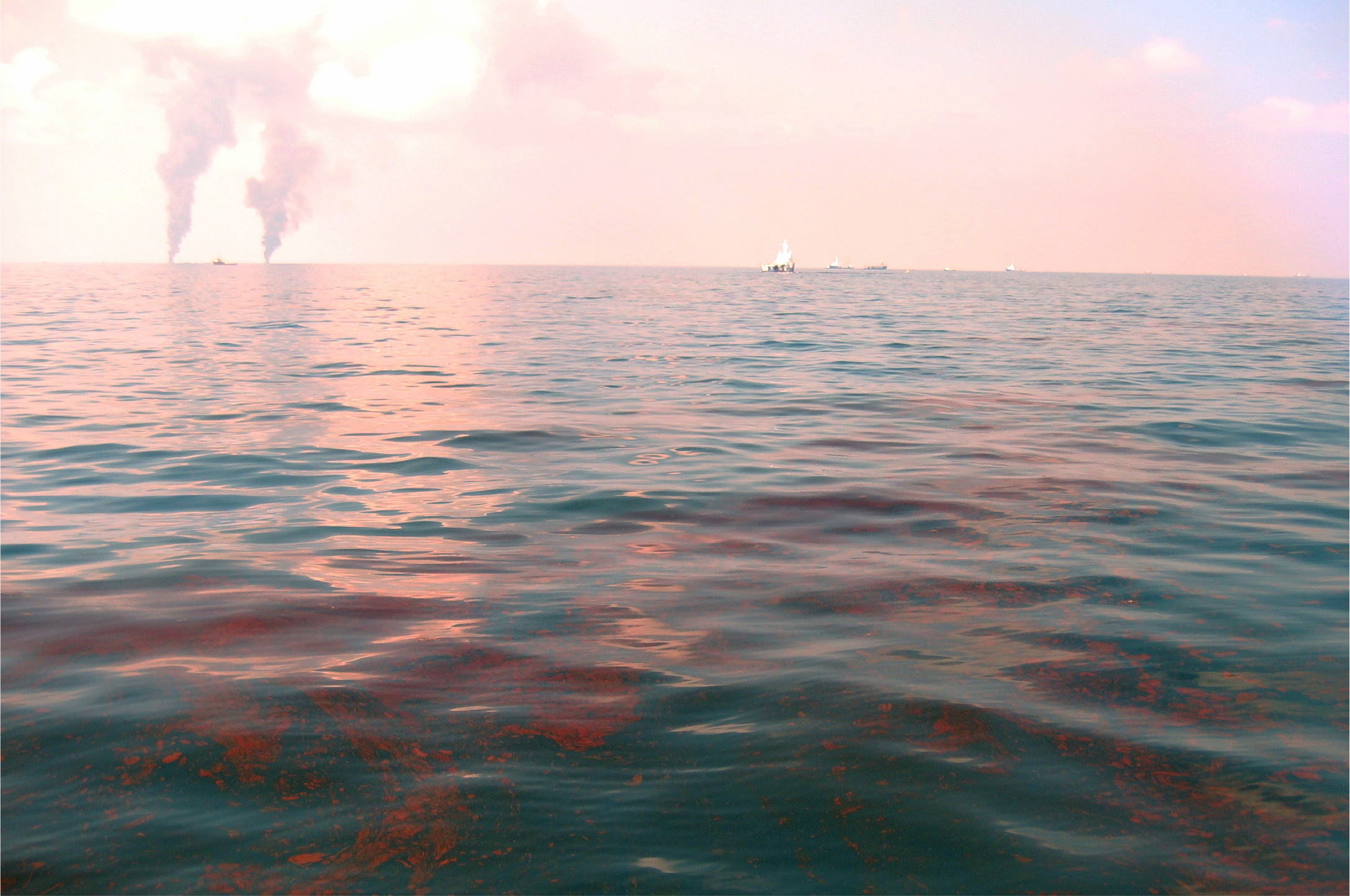
Figure 4. This image from a Gulf of Mexico research cruise in the spring/summer of 2010 captures in situ burning and mechanical recovery of oil at sunrise and illustrates the challenges of capturing and collecting all of the surface oil. Photo credit: Samantha Joye, University of Georgia. > High res figure
|
GoMRI and NRDA research provided new insights on water column and benthic area impacts of oil dispersed physically and chemically by subsea dispersant injection. Murawski et al. (2019) consider conflicting theories on the effectiveness of subsea injection. While there are still research gaps and political implications regarding the use of dispersants, to properly evaluate their use, response leaders will still need real-time predictive modeling of the fate and transport of oil that cannot be mechanically collected. In addition to GoMRI research, other efforts have also tried to address dispersant use (NASEM, 2020) but need to include the “what if dispersants aren’t used” or “what if burning isn’t used” impacts to and recovery times of nearshore and coastal environments.
Although modeling, as described above, along with a method for comparative risk assessment (French-McCay et al., 2018; Bock et al., 2018; and A.H. Walker et al., 2018), is beginning to address these questions, a fully developed and “agreed upon” systems dynamics model does not yet exist. It is challenging, if not impossible, to develop a value system where loss of deep-sea corals and closure of offshore fisheries could be weighed against the impacts to nearshore habitats and tourism, but during an event, those decisions must and will be made by the Incident Command. As we continue to improve our data collection and modeling, scientists need to work through the various scenarios and help lead the discussion of complex trade-offs that will continue to improve comparative models as they relate to response planning and operational decision-making.
Freshwater Diversions and the Complexity of Evaluating Operational Alternatives
Future spills will inevitably present response leadership with novel spill conditions, requiring that alternative response approaches be quickly evaluated. Although an established protocol for this evaluation considers potential success, feasibility, and impacts, trying new methods or untested equipment can have adverse impacts and increased liability. During DWH, numerous alternatives were offered for evaluation, and some were undertaken, including freshwater diversion and construction of sand berms. The freshwater diversion of Mississippi River waters, which was designed to minimize damage to coastal wetlands by keeping oil offshore, demonstrated a trade-off decision that put tremendous stress on other parts of the ecosystem. Equally important is applying the same rigorous trade-off decision to any restoration project that might be funded through damage assessment or other restoration efforts. This could incorporate even more complex processes associated over time with nutrient overloads, land use management, climate change impacts, natural erosion, and future energy development.
During DWH, several ad hoc countermeasures were employed to forestall oil coming ashore or entering sensitive wetlands where there would be significant damage and difficult remediation. Coastal defense measures such as the construction of sand berms around islands (e.g., Suir et al., 2016) and increasing flow rates of Mississippi River water diversions were implemented in an effort “to try and push the oil away from our coastal wetlands” (State of Louisiana, 2010). The concept of using existing river diversions to create elevated coastal flows and thereby divert surface oil was simulated in late April 2010 by the US Army Corps of Engineers Engineering Research Development Center using their Adaptive Modeling System. These simulations evaluated the hydrology resulting from increasing flows through the Davis Pond Diversion (upstream from New Orleans) and the Caernarvon Diversion (Downstream of New Orleans) to flood marshes of Barataria Bay and Black Bay/Breton Sound, respectively (Figure 5; USACE, 2010). Results of these simulations were promising, and during the first week of May 2010, flow rate discharges were increased dramatically through these two structures and a number of other smaller diversions (Figure 6; State of Louisiana, 2010; O’Connor et al., 2016).
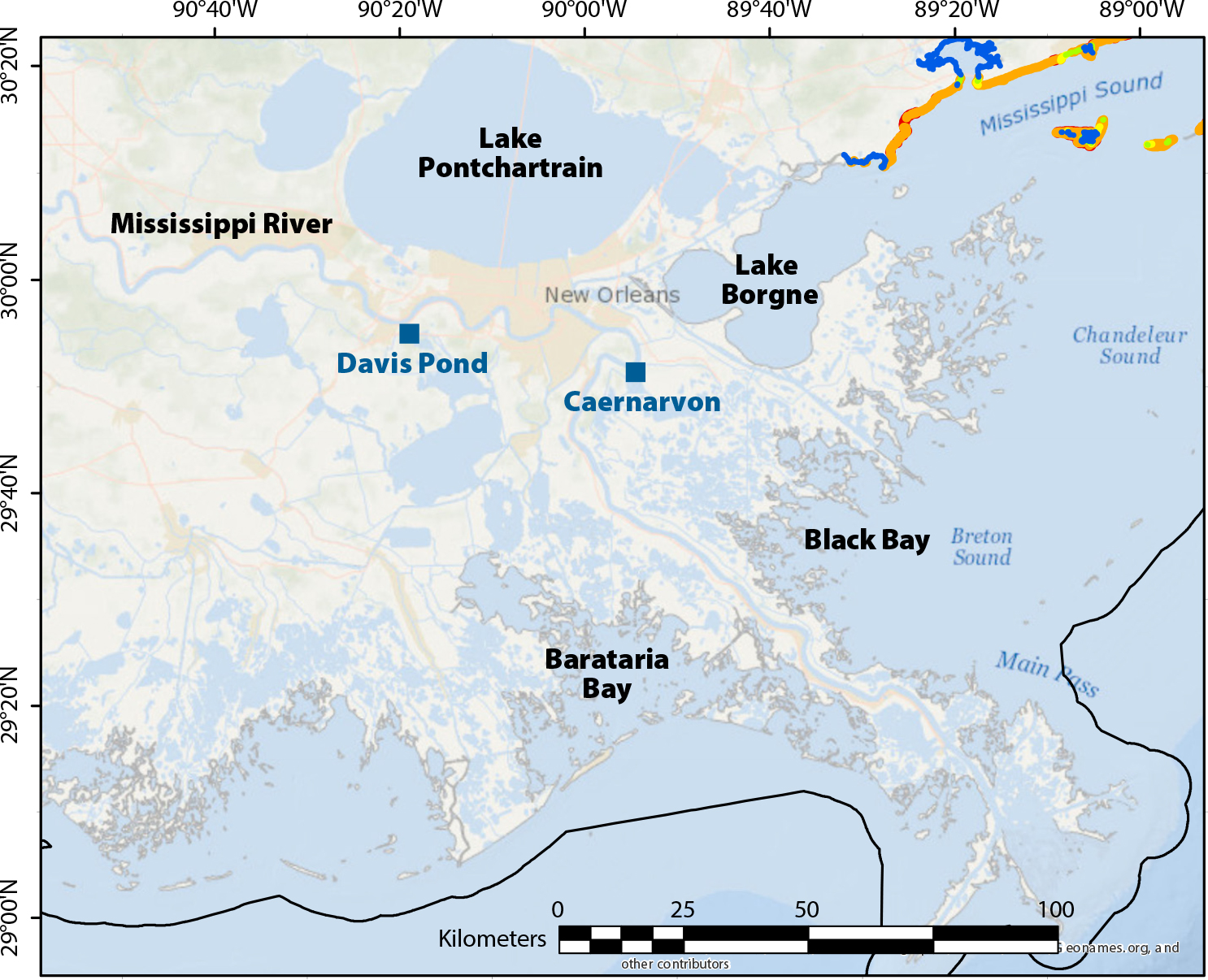
Figure 5. Locations of the Davis Pond and Caernarvon water diversion structures along the Mississippi River. The black line is the three-mile state territorial boundary. > High res figure
|
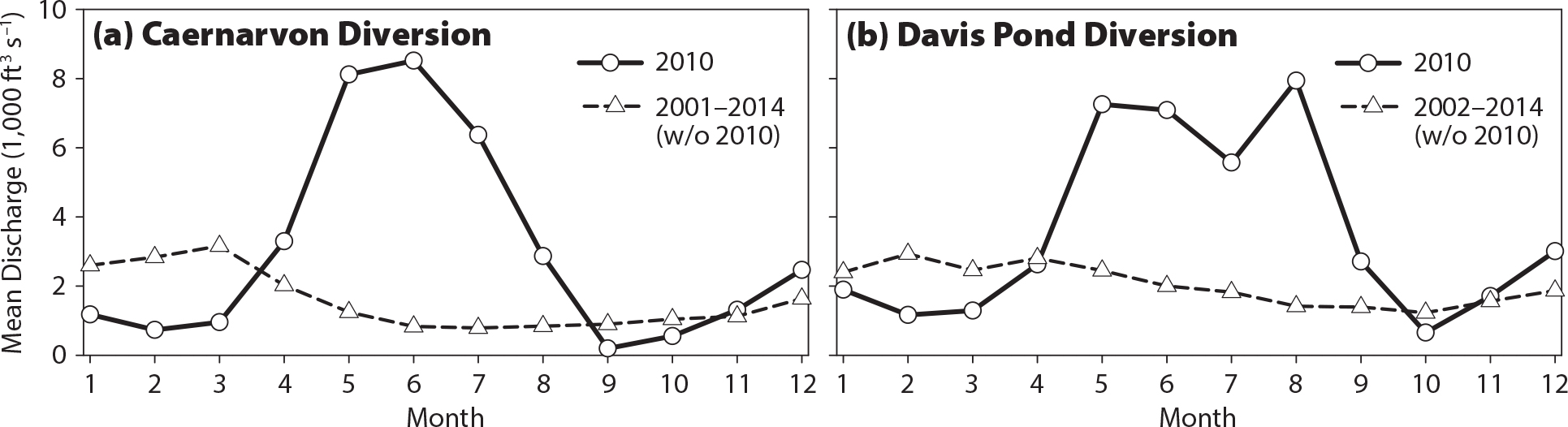
Figure 6. Mean discharge rates (1,000 ft3 s–1 or 28 m3 s–1) from Mississippi River diversions at (a) Caernarvon and (b) Davis Pond for 2010 and mean 2001–2014 without 2010 for Caernarvon, and 2002–2014 without 2010 for Davis Pond. Data are found at https://nwis.waterdata.usgs.gov/usa/nwis/. > High res figure
|
In the case of the Davis Pond Diversion, flow rates were increased to about three times the pre-2010 average, and for Caernarvon, about eight times normal flow (Figure 6; O’Connor et al. 2016). While the degree to which these elevated freshwater discharges affected oil transport into these bays is conjectural, they were not completely effective, as oil concentrations within the bays reached over three orders of magnitude greater than background contamination in some locations (Turner et al., 2019a) and remained several orders of magnitude higher than baselines for at least five years thereafter (Duan et al., 2018). Model simulations have shown that elevated diversion discharges have no influence on hydrodynamics >30 km from the diversion sites and are not effective in preventing offshore oil slicks from drifting into Barataria Bay and Breton Sound estuaries (e.g., Huang et al., 2011).
The effects of diversions are closely linked to the dynamics of river plumes and the overall coastal and shelf processes that influence the transport and fate of materials (e.g., sediments, nutrients, oil) that are either river-borne or entrained on the shelf areas from offshore sources. Large rivers such as the Mississippi create their own offshore circulation, driven by the buoyancy of the fresher waters and mediated by winds and topographic controls (Androulidakis et al., 2015). In the Northern Gulf of Mexico, a unique effect is that the river-induced circulation can be heavily influenced by offshore currents, specifically the Loop Current and its associated eddies (N.D. Walker et al., 2005; Schiller and Kourafalou, 2014), causing a possible advection of river-borne materials far away from the freshwater discharge sites (N.D. Walker et al., 1994; Schiller et al., 2011; Androulidakis et al., 2019). Therefore, the amounts of riverine waters and materials that might remain on the shelf do not solely depend on the amount of river discharge that can be controlled through diversions (e.g., Androulidakis and Kourafalou, 2013; Le Hénaff and Kourafalou, 2016).
Initial simulations conducted by the US Army Corps of Engineers evaluated the hydrologic effects of diversions on marsh flows but did not assess the degree of change in the salinity gradients across marsh landscapes nor the effects of such alterations on ecosystems. Considerable research conducted contemporaneously with the diversions documented sustained reductions in salinity and concomitant impacts on sessile euryhaline species including the eastern oyster (Grabowski et al., 2017; Powers 2017a,b) and other species. While resident marsh species such as oyster are extremely tolerant of normal variations in salinity and other physical attributes, sustained periods of very low salinities will result in mortalities. Powers et al. (2017b) estimated that billions of excess mortalities of oysters occurred because of sustained (>50 days) low salinity (<5 ppt) conditions that resulted from the water diversions associated with DWH. They succeeded in disentangling the impacts on oyster mortality due to low salinity from those due to oiling and concluded that there was no discernible relationship between oiling and elevated oyster mortality, but rather mortalities were due to sustained freshwater conditions.
In contrast to oysters, salt marsh fish assemblages (e.g., killifishes and associated species) were relatively unaffected by the spill and changes in the salinity regimes (Able et al., 2014), primarily because these species are adapted to locations (salt pans and upstream marshes) that naturally exhibit extremes in temperature, salinity, and water levels. However, for the community of fishes and invertebrates occupying the open estuary in Barataria Bay, the region most affected by wetlands oiling (Nixon et al., 2016) and subject to significant freshwater diversions, there was a regime shift in the abundance and species composition of trawl catches that has persisted post-spill and is likely associated with decreased salinity and increased water clarity since the spill (Murawski et al., 2021b).
The impacts of the 2010 diversions could have been predicted based on the wealth of pre- and post-construction ecological studies conducted for the Caernarvon diversion (see Chesney et al., 2000, for a summary). Numerous studies conducted prior to the 1991 opening of Caernarvon and the 2002 opening of Davis Pond, and undertaken since DWH, point to shifting of the salinity gradients, potential increases in freshwater species such as bass and catfish, downstream movement of key estuarine fish species, and reduced oyster survival in prime habitats (Das et al., 2012; de Mutsert and Cowan, 2012; Rose et al., 2014; Powers et al., 2017a). During and after the DWH episode, oiling of bottlenose dolphin populations resulted in severe negative health effects and elevated mortalities (Schwacke et al., 2014, 2017). As dolphins are intolerant of extended periods in fresh waters, there is an open question regarding the role that lower salinities may have played in concentrating dolphins near passes and inlets where the DWH oil entered marshes (White et al., 2018).
Given the tremendous investment in additional permanent, large-scale diversions of Mississippi River water and sediments into Barataria Bay and Breton Sound (Coastal Protection and Restoration Authority of Louisiana, 2017), we can anticipate that many of the ecological changes resulting from the experimental use of elevated discharges during DWH will be played out in the long term. The intentions of these planned diversions are to create additional sediment substrate supporting fresh and brackish marshes and to reclaim wetlands habitats from open waters. Documenting such effects from these diversions and other parallel engineering projects will be critical in justifying their enormous expense—estimated to be over $50 billion (Coastal Protection and Restoration Authority of Louisiana, 2017). With respect to the use of water diversions as a countermeasure for future oil spills, we can conclude that in the case of DWH they were ineffective in achieving their primary objective, to “try and push the oil away from our coastal wetlands,” and that doing so resulted in unanticipated, severe negative ecological effects.
Research Priorities
The use of freshwater diversions during the DWH event and their ecological impacts highlight important research gaps that should be closed, not only if diversions are once again considered as oil spill countermeasures, but primarily to better understand the consequences of additional large-scale diversions planned as restoration activities, supported in part by DWH settlement money. While it is clear that these efforts are directed toward ecosystem improvement, the effectiveness of the diversions and other measures in creating habitat substrate has been questioned (Teal et al., 2012; Turner et al., 2019b). Likewise, by developing a firmer understanding of how ecological processes and individual species will react to both the acute effects of the diversions and the long-term consequences of habitat alterations, it may be possible to optimize the operation of the diversions to better achieve multiple natural resource goals. It will be critical to:
- Monitor the long-term impacts of DWH on marine and estuarine habitats affected by freshwater diversions and those planned as part of large-scale restoration activities. Monitoring should be used in an adaptive management approach to minimize negative ecological effects and maximize potential to meet the prime objective (substrate creation).
- Understand the movement ecology of freshwater, estuarine, and marine species likely to be affected by the operation of current and planned diversions to parameterize predictive models of ecosystem response. These studies could include an intensive network of tag sensors to track the movements of individuals and allow determination of the connectivity/modularity of key living resources and their roles in ecosystem resilience and recovery.
- Develop new classes of predictive numerical models to explore the effects of planned large-scale freshwater diversions. Such models would integrate estuarine, coastal, and shelf hydrodynamics and incorporate ecological processes and outcomes, thus allowing for better predictions of the outcomes of long-term habitat restoration activities over decadal to century scales and also of their short-term effectiveness in oil spill countermeasures.
The Future
Oil spill research after DWH resulted in improvements in the tools needed to guide operational response, but continuing this effort will be dependent on dedicated effort and funding. Questions still remain, and new questions and opportunities need analysis. New technologies may be useful in future spill responses, but they too could produce unintended impacts. A few of these include remote deployment of surface sensors that feed immediately into models and of untethered sensors to obtain droplet size distribution and other data at depth, introduction of genetically modified microbes to enhance biodegradation, use of oil herders and enhanced burn technologies, new formulations of dispersants, increasing use of genomics in damage assessment, and deployment of unmanned boats and aircraft that could manage mechanical equipment and alternative countermeasures. DWH and associated funding provided a rare opportunity for researchers across disciplines in industry, government, and academia to work with and improve plans, preparedness, and response. Because oil spills will continue to occur, research funding and academia’s connection to operational decision-makers must continue to be fostered in order to close gaps, evaluate new opportunities, and bring forward the best science for application to spill response plans and operations.