The View from Above: Observations of Surface Oil
Important advances have been made in ocean observing through both in situ and remote-sensing technologies that include drifters, satellite and aircraft sensors, and high-frequency ocean radar (HFR) systems, tools that are especially useful for determining transport at and immediately below the ocean surface (Morey et al., 2018; Rodríguez et al., 2018). Data collected using these methods show that the velocities measured right at the ocean surface, as opposed to many centimeters or meters below it, are much greater than previously thought, and these differences need to be accurately represented in models (Le Hénaff et al., 2012; Wenegrat et al., 2014). From April 22 to August 2, 2010, every available satellite was tasked with observing the northeastern Gulf of Mexico at every possible opportunity. Their collective mission was to detect floating oil that reached the ocean surface from wreckage of the Deepwater Horizon (DWH) rig and the blowout of the exploration well in the Macondo MC252 lease block. The effort yielded an unprecedented data set comprising over 200 optical and 600 synthetic aperture radar (SAR) scenes that imaged portions of a surface oil layer (slick) that covered an average of 11,200 km2 (sd 5,028) for 87 days. The slick’s “footprint” changed on an hourly basis as the oil drifted with wind and currents, sank and resurfaced, landed on the Gulf coast, and ultimately dissipated.
This summary focuses on results from analysis of 166 of these high-resolution SAR images of small-scale sea surface elevation and roughness conducted under the auspices the NOAA National Resource Damage Assessment (NRDA) program, with additional support from the Gulf of Mexico Research Initiative (GoMRI) ECO-system impacts of fluid and Gas Inputs from the Geosphere (ECOGIG) I and II consortia (MacDonald et al., 2015). Satellite SAR images detect floating oil layers because oil suppresses small capillary and gravity waves, thus creating a smooth surface that deflects radar energy more effectively than does surface water that is free of oil. These oil layers are imaged as dark patches that contrast with the unoiled sea (Garcia-Pineda et al., 2013b). The contrast is most visible when wind speeds are moderate, in the range of 2–10 m s–1 (Daneshgar Asl et al., 2017). Unlike optical sensors, satellites with SAR detectors function irrespective of cloud cover or time of day (Leifer et al., 2012). The SAR scenes used in the study originated from Radarsat-1, Radarsat-2, TerraSAR-X, CosmoSKY-MED 1-2-3-4, ENVISAT, ALOS-1, and ERS-2. The resolution of this large SAR data set ranged from 10 m to 25 m per pixel over 25 km to 450 km swaths. The Gulf of Mexico region where oil was detected in each SAR scene was gridded at a 5 km scale so that results could be summarized across large ocean areas in a time lapse throughout the duration of the spill (Holmes et al., 2017).
The NRDA team developed two innovative approaches to further analyze the data. While each scene generally covered hundreds of square kilometers, the total extent of the floating oil was often much greater in area; thus, each image captured only samples of the larger phenomenon. To obtain an interpolated and gridded time series showing the areal extent of the entire oil slick at 12 h intervals, MacDonald et al. (2015) authors Solow and Beet developed a statistical method in which the oiled areas detected in each SAR scene were treated as unbiased samples of the total oil layer. Images of floating oil slicks were highly patchy and heterogeneous in apparent thickness. To estimate the volumes of oil represented by different portions of the oil layer, Garcia-Pineda et al., 2013a,b) developed image-processing methods that analyzed the signal strength of SAR pixels to distinguish between “thin” or “thick” layers (nominally 1 μm and 72 μm, respectively).
Results could be interpreted in relation to environmental factors (e.g., wind speed), the rate of discharge from the well, and in the context of response operations such as surface oil burning and application of dispersant by aerial and submarine methods. Figure 1 shows the average extent of surface oil in units of m3 km–2. An animation of the 12 h estimates, augmented with data wind speed can be viewed at https://www.youtube.com/watch?v=v1kkniG7atA&t=4s, and original data can be retrieved from the GRIIDC database (MacDonald, 2019).
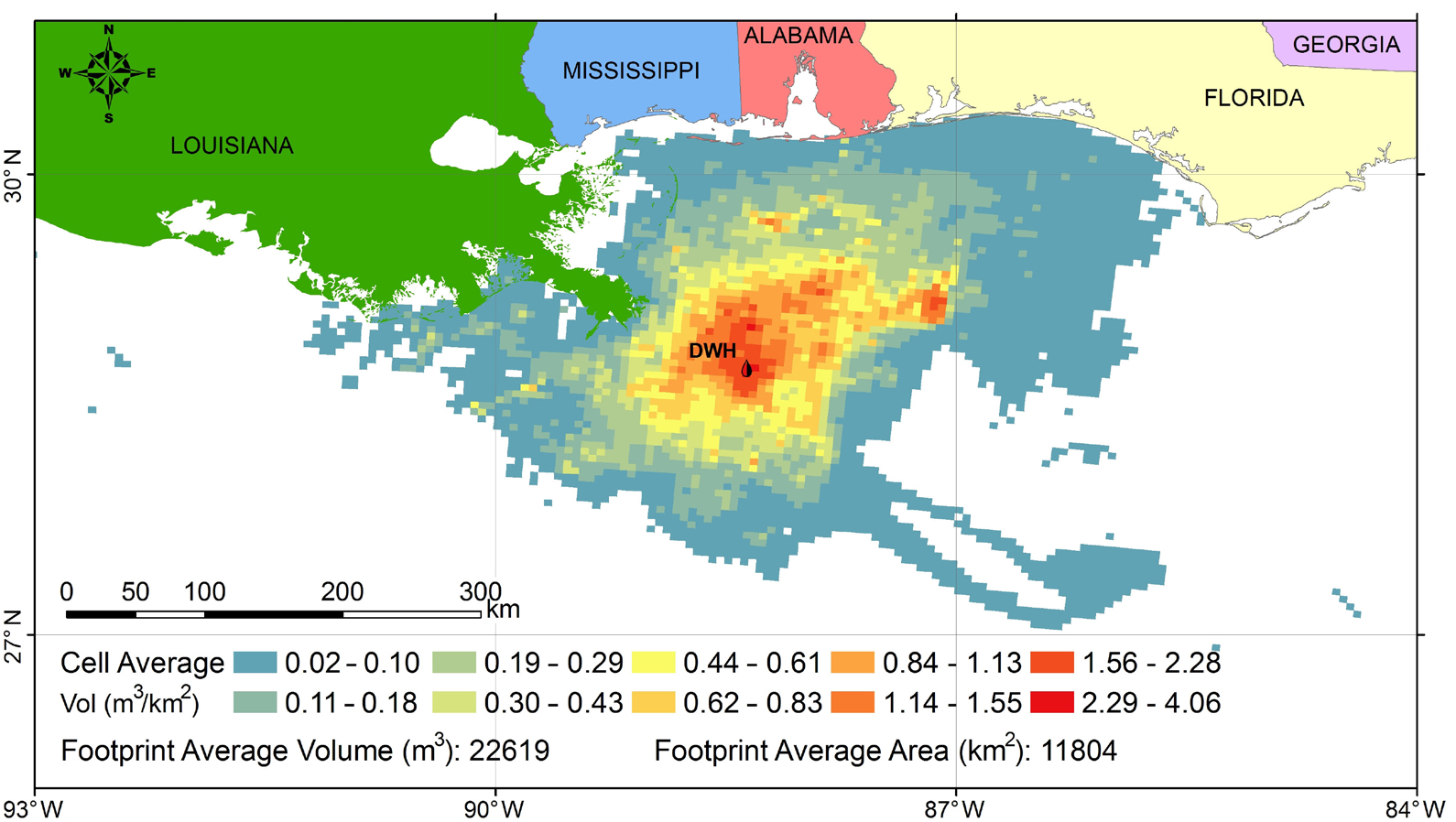
Figure 1. Distribution and average volume of surface oil (m3 km–2) from the Deepwater Horizon (DWH) discharge, gridded at 5 × 5 km scale across a cumulative footprint of 149,000 km2, from April 24, 2010, to August 3, 2010. From Figure 3 in MacDonald et al. (2015). > High res figure
|
The unprecedented remote-sensing data set has been used not only to better understand the extent and impact of the DWH spill but also to develop better methods and technologies for detecting oil spills. For example, during the spill in 2010, it could take several days to provide spill responders with a processed satellite image; this process has now been narrowed to a matter of minutes. In parallel, methods for extracting more detailed characterizations of the floating layers of oil from SAR satellite images (emulsification, thicknesses, and/or discerning false positives) have also been substantially improved.
The findings from this study have been used in a number of subsequent investigations, including how floating oil impacts benthic deposition by marine snow (Daly et al., 2016); the responses of the microbial community to the spill (Joye et al., 2016b); the spill’s impacts on seabirds (R.M. Huang et al., 2017), fishes (Price and Mager, 2020), and cephalopods (Romero et al., 2020); and the extent of fishery closures (Berenshtein et al., 2020). One novel result concerned the possible impact of dispersant applications. There were two roughly equivalent periods of the oil spill during which winds were low and oil slicks could spread with reduced mixing from wave action: peaks in the total area and the volume of the floating oil layer were observed on May 23 and June 18, 2010. Between these peaks, the total volume of floating oil decreased by 21%, while the ocean area over which the floating oil spread increased by 49% (Figure 2). On approximately June 3, responders began application of up to 50,000 L d–1 of Corexit dispersant directly into the discharging oil plume at depth (Lehr et al., 2010). Thus, the satellite data suggest that the submarine dispersant application may have achieved a reduction in the total volume of surface oil, but with the side effect of increasing the area over which the residual volume was dispersed. In addition, after June 1–3, the damaged end of the riser of the well was cut off, and a new cap/collection system was installed that siphoned off ~15,000 barrels per day, or 20%–25% of the oil released (Griffiths, 2012), also contributing to the measured decrease in floating oil. It is hoped that this experiment in satellite remote sensing of an oil spill will not be repeated, but the data obtained were and will remain an important resource for understanding the dynamics of floating oil in the marine environment.
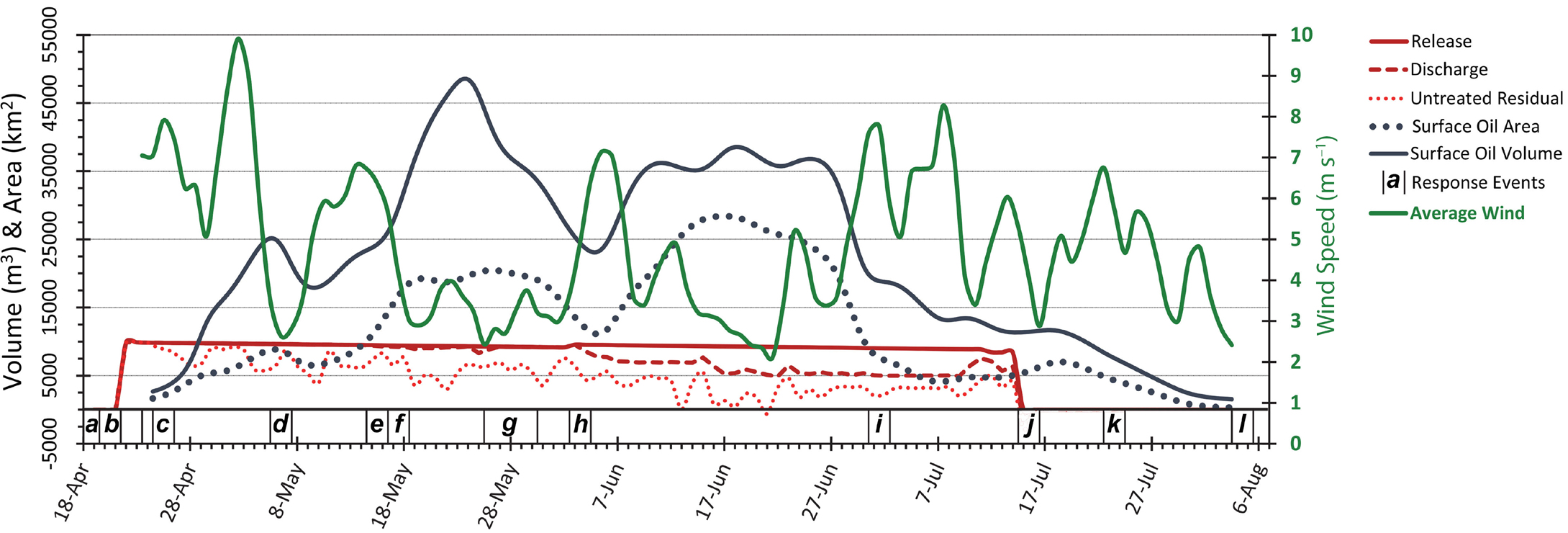
Figure 2. Time series of DWH discharge during 2010, plotted with surface oil and average wind speeds. Release magnitudes show best daily estimates of oil escaping from the damaged well. Discharge subtracts the oil recovered from the gross release, while treatment further subtracts that burned and dispersed by aerial and subsea applications of Corexit at maximum efficacy. Response events that potentially affected the spread of surface oil are: (a) Macondo well blowout occurs, (b) DWH drillship sinks and release begins, (c) aerial dispersant application begins, (d) containment dome attempt fails and burning of surface oil begins, (e) subsea dispersant campaign begins (May 5), (f) flaring of recovered oil begins, (g) top-kill attempt made, (h) riser is cut from blowout preventer and direct oil collection subsea and injection of subsea dispersant begins, (i) Hurricane Alex makes landfall, (j) capping stack closure stops release, (k) Tropical Storm Bonnie makes landfall, and (l) well is killed by static backfill. From Figure 7 in MacDonald et al. (2015). > High res figure
|
Microscale Physical (and Biophysical) Processes
GoMRI research included a series of studies focused on measuring and modeling the very small-scale physical and chemical processes that affect the interactions of oil with the marine environment. These investigations include: (a) physical breakup of oil slicks by waves and the associated effects of dispersants; (b) measuring and modeling the breakup and droplet generation in submerged oil jets and plumes; (c) applications of Large Eddy Simulations (LES) to simulate coherent oil plumes in the marine environment; (d) characterization of interfacial phenomena involving oil, dispersants, and bacteria, and mass transport at oil-water interfaces; and (e) phenomena involving the aerosolization of oil. All of these aspects are discussed below.
Breakup of Oil Slicks
Wave tank measurements (C. Li et al., 2017; Afshar-Mohajer et al., 2018) provide a comprehensive database on the spatial and temporal size distribution of subsurface droplets as a function of wave energy, dispersant concentration, oil viscosity, depth, and time after wave breaking. The measured time evolution of turbulence in the tank has been used for correlating the evolution of droplet size distribution to their subsurface transport. As expected, for fresh crude oil without dispersant, after wave breaking, the measured rate of droplet disappearance from the water column increases with buoyancy and with droplet size. This can be predicted based on a solution to an unsteady advection–diffusion (turbulent) equation, and the characteristic droplet sizes are consistent with models involving turbulent Weber numbers.1 The results have subsequently been used for validating predictions of wave breaking and for modeling oil transport after spills, and also to support parallel numerical simulations and characterization of chaotic phenomena in wave breaking (Wei et al., 2018). Application of dispersant (Corexit 9500) causes major changes in droplet size distributions, reducing the concentration of millimeter-size droplets and increasing the concentrations of microdroplets. For a dispersant-to-oil ratio (DOR) of 1:25 in particular, the micron-scale droplets are generated by an interfacial phenomenon called tip streaming (Gopalan and Katz, 2010). This phenomenon involves generation of long oil strings that extend from separation points on the surface of the droplet. The Weber-number-based predictions of droplet sizes do not account for these interfacial phenomena, and hence they overpredict droplet sizes. A computational study aimed at elucidating the causes for tip streaming (Tseng and Prosperetti, 2015) shows that it is generated by an instability that occurs near special points on the interface. Wherever the flow at the interface is locally convergent, any small perturbation on the interface tends to grow because of the combination of compression and viscous shear stress. The effect of surface tension opposes this growth, but its influence is inhibited in the presence of surfactants, especially because the convergent flow also accumulates the surfactants in its vicinity.
1 The Weber number describes the magnitude of the hydrodynamic forces that tend to disrupt droplets, relative to the surface tension that tends to stabilize them.
Droplets in Oil Jets and Plumes
Considerable effort has been made to measure and model droplet size distributions in jets and plumes. Relying on classical turbulent models, Johansen et al. (2013) introduced a semi-empirical Weber-number-based model for the size of oil droplets in jets that has been subsequently updated using experimental data (Brandvik et al., 2013, 2014; Johansen et al., 2013, 2015). They also characterized depth effects (Brandvik et al., 2019), and introduced new methods for classifying oil droplets, particles, and bubbles based on their quasi-silhouette images (Davies et al., 2017). Corrections for large jets, which account for the maximum possible droplet size, are discussed by Z. Li et al. (2017a,b). These models also account for the effect of dispersant through reduction of the interfacial tension, but not for the interfacial processes, such as tip streaming, which is believed to be responsible for generating micron-size droplets Murphy et al., 2016; Zhao et al., 2017). Another approach, the VDROPJ model (Zhao et al., 2014a) uses the droplet population dynamical model VDROP (Zhao et al., 2014b) and applies it to control volumes in the near fields of the jet/plume, thus allowing for changes in jet hydrodynamics to impact the size distribution. VDROP allows for breakup and coalescence of droplets, accounts for resistance to breakup by interfacial tension and viscosity, and includes modules that simulate tip streaming. While the microdroplets account for only a small percentage of the total oil volume, they are more likely to be entrained into the intrusion layers (D. Wang and Adams, 2016; Gros et al., 2017), whereas larger droplets (e.g., >500 µm) tend to rise directly to the surface (Zhao et al., 2015).
Murphy et al. (2016) describe droplet sizes and spatial distributions and the flow mechanisms affecting them in the near field of oil jets in realistic cross-flow conditions. They show that the bottom part of the oil plume near field is dominated by entrainment of droplets into the counter-rotating horizontal vortex pair set up by the cross flow, while the upper part is determined by the buoyant rise of relatively large droplets. Because the plume structure is strongly influenced by the droplets’ size distributions, subsequent studies have focused on the breakup of vertical oil jets into droplets. Observations involving refractive index matched jets (Xue and Katz, 2019) show that most of the oil droplets are actually compound droplets; they contain smaller water droplets, which sometimes contain even smaller oil droplets. The fraction of compound droplets increases with droplet size, exceeding 80% for 2 mm droplets. These compound droplets are generated when early phase shear layers roll up into vortices and entrain water filaments into the oil.
Computational Modeling of Plume Behavior
Studies of plume dispersion are challenging because of the multiscale flow physics involved, ranging from individual oil droplets to an entire plume and to mesoscale eddies in the upper ocean boundary layer. Applications of LES2 methods to characterize such flows require parameterization of the subgrid-scale processes that are not resolved, including oil droplet and gas bubble formation, coalescence and breakup, gas dissolution, biodegradation, and hydrate formation. Several LES-based studies of oil and gas plumes have investigated the effect of droplet-scale physics on the plume-scale dispersion phenomena (Yang et al., 2016; B. Chen et al., 2016b, 2018). Among the many findings, they show that: (1) the significant reduction of oil droplet size caused by dispersant also enhances the vertical mixing of the oil in the ocean mixed layer, resulting in an Ekman-spiral-induced shift of the mean plume transport direction; (2) application of a recent droplet population balance model (Aiyer et al., 2019) in an LES framework (Chamecki et al., 2019) to simulate the effect of turbulence on oil droplet breakup reproduces the measured droplet size distributions (Murphy et al., 2016); (3) LES of subsurface plumes shows that gas dissolution from bubbles causes a considerable reduction in plume buoyancy and impacts the peeling/intrusion processes. A related theoretical study investigated the dissolution of multi-component oil drops in water, where the surface concentration of solutes depends on the drop composition and varies as the drop dissolves due to the different solubilities of individual components (Chu and Prosperetti, 2016). The analysis involves a first-principles demonstration of the necessary modeling strategy based on the fundamental law of equality of each component’s chemical potentials in the drop and in the external solvent.
2 Large Eddy Simulation (LES) methods deliberately filter out very small-scale motions, which are the most computationally expensive to resolve, producing results that are smoothed somewhat in both space and time but that preserve the larger scale features.
Interactions Between Microbes, Particles, and Oil Droplets
A series of experimental studies have focused on micrometer-scale interactions between microbes, particles, and oil droplets (Sheng et al., 2006; Molaei and Sheng, 2014; Jalali et al., 2018; White et al., 2019a). The degradation of 5–100 µm droplets through dissolution and biodegradation by bacterial isolates and natural microbial consortia, with and without dispersant, was measured using microfluidic flows over a surface textured with microdroplets (Jalali et al., 2018). For 100–1,000 µm droplets, the experiments were performed in a unique ecology-on-a-chip microcosm platform that allows examination of bacterial behavior and microbial community responses around a rising micro-oil droplet, along with measurements of associated flows and rheological mechanisms (White et al. 2019a,b). These studies demonstrate the formation of polymeric microbial aggregates as well as extra-cellular polymeric substance (EPS) streamers around rising oil droplets, both of which affect the droplets’ hydrodynamic impacts—even a few isolated streamers can increase the drag on a drop by 80%. Another study of bacterial interactions with alkane-aqueous interfaces (Vaccari et al., 2017) shows that these interactions depend strongly on the particular strain of bacteria. The bacteria can either continue to swim adjacent to the interface or become attached to it. If they become attached, they may form a thin solid elastic film (Vaccari et al., 2015). Some, but not all, of the observations suggest that the ability to form such elastic films relies on the ability of the bacteria to metabolize the oil phase (Niepa et al., 2017). Bacteria near interfaces display a range of behaviors that includes Brownian motion, swimming in circular trajectories, and directed swimming (Deng et al., 2020). These motions can also enhance the dispersion and transport of micron-scale droplets (Vaccari et al., 2018).
Aerosolization of Oil
Surface oil slicks can be aerosolized either by splashing during wave breaking or by bursting of entrained bubbles as they rise back to the surface. While the size distributions of marine aerosols have received considerable attention (Veron, 2015), very limited information exists about the process of aerosolization of oil slicks. However, a series of experiments investigated the effect of dispersants on the aerosolization of oil slicks by breaking waves (Afshar-Mohajer et al., 2018), and subsequently, the effect of bubble bursting at an oil-water interface (Sampath et al., 2019; Afshar-Mohajer et al., 2020).
In these wave tank experiments (Afshar-Mohajer et al., 2018), the aerosol size distributions were measured in sizes ranging from 10 nm to 20 µm using a scanning mobility particle sizer (SMPS) for the 10–400 nm size range and an aerodynamic particle sizer (APS) for the 0.5–20 µm range. Digital holography (Katz and Sheng, 2010; C. Li et al., 2017) has also been used in parallel for detecting airborne droplets larger than 4 µm, showing good agreement with the APS data. The total particle-bound polycyclic aromatic hydrocarbons (pPAH) and the total volatile organic compounds (TVOCs) were measured concurrently. Data have been obtained for plunging breaking waves entraining slicks of crude oil (MC252 surrogate), crude oil-dispersant mixtures, and dispersant only. The results show that slicks of crude oil do not alter the concentration of nanodroplets. In contrast, the concentrations of nanoparticles originating from oil-dispersant mixture and dispersant alone are one to two orders of magnitude higher than those from crude oil alone across the entire nano-scale range, reaching 100 times for 20 nm particles. Conversely, adding dispersant has little effect on the concentration of micro-aerosols. The average concentrations of pPAH remain similar in range (150–270 ng m–3). However, the VOC concentrations for crude oil-dispersant mixtures are two to three times lower than those of crude oil, in agreement with prior studies (Gros et al., 2017). An analysis of the health risk associated with the measured VOC and airborne particles is discussed in Afshar-Mohajer et al. (2019). Their results show that on the one hand, the lower VOC reduces the health risk. On the other hand, inhalation of the nanoparticles increases the total mass of deposited particles in the upper respiratory tract and tracheobronchial regions of the lungs, although the measured quantities were still well below the health risk threshold. However, the concentration of total suspended particles (micro plus nano scale) close to the source only just exceeds the hazard threshold level by 1.1 times for crude oil without dispersant, but by 6.8 times for crude oil premixed with dispersant.
In an attempt to identify and characterize the mechanisms affecting the generation of nanodroplets, subsequent studies focused on generation of airborne droplets by bursting of rising gas bubbles at the liquid-air interface (Sampath et al., 2019). Bubbles with different size ranges were injected into a seawater column covered by slicks of crude oil, pure dispersant, and a DOR 1:25 dispersant-oil mixture; the sensors mentioned above were used to measure nano- and micro-aerosol size distributions in both clean and ambient air environments. An order of magnitude increase in nanoparticle concentration was observed during bursting of bubbles larger than about 0.5 mm in slicks containing dispersant at a DOR of 1:25 of oil, and for a 50 µm layer of pure dispersant, compared to smaller bubbles and tests not involving dispersant. These observations suggest that of the two mechanisms causing generation of airborne droplets during bubble bursting, namely jet droplets and film droplets (Veron, 2015), the nano-aerosols originated from breakup of the thin water film above the bubbles. In the micron range, all the bubble plumes generate micron-sized aerosols, but trends vary with bubble size and slick thickness, and the presence of dispersant does not cause an increase in the concentration of micro-aerosols compared to that for dispersant-free conditions. Based on their characteristic sizes, the micro-aerosols originated from jet droplets. In general, for the same surface contaminant, the microdroplet concentration decreases when the oil slick is thicker. A reduction of two orders of magnitude in the microdroplet concentration when medium and small bubbles burst in a thick (500 µm) surface slick of crude oil is particularly striking.
Another study involving slicks of crude oil and oil-dispersant mixtures at DORs of 1:25 and 1:100 focused on the chemical composition of airborne PM2.5 particles (Afshar-Mohajer et al., 2020). These measurements included chemical analysis by gas chromatography and mass spectrometry, as well as use of a cascade impactor for size-fractioning of the particles. Results show that the total PM2.5 concentration released from the DOR 1:25 slick is about nine times higher and the oil content in them is about 2.4 times higher than those for pure crude oil. For particles smaller than 220 nm, the crude oil concentrations for DOR 1:25 and 1:100 are about six and three times higher, respectively, than those for pure crude oil.
Several other studies involving aerosolized oil should also be mentioned. First, the generation of airborne droplets when raindrops impact oil slicks is discussed in Murphy et al. (2015). They show that the presence of oil slicks and dispersant alter the splashing and aerosol generation processes, and that the number of microdroplets increases both with oil layer thickness and with addition of dispersants. Second, M. Li et al. (2019) apply a wave-effect-resolving LES to simulate the transport of oil droplet aerosols by wind over surface waves in the marine atmospheric boundary layer. They show that wind considerably enhances oil droplet suspension and both vertical and lateral spreading, and that the spatial distribution of aerosols exhibits significant streamwise variations. Finally, Almeda et al. (2018) show that oil spills and dispersants can increase harmful algal blooms (HABs) and their associated toxins in the upper water column. Hence, in addition to risks associated with aerosolization of oil, changes to the resulting airborne toxins should also be considered if and when HABs are present.
Near-Field Processes: Plume Formation and Dynamics
Above the primary bubble and droplet breakup zone, a buoyant plume of gas, oil, and entrained water forms and rises through the density-stratified ocean water column (Socolofsky et al., 2016; Boufadel et al., 2020). Depending on the water depth and stratification, the plume may pass through a level of neutral buoyancy, be arrested in its vertical ascent, and form a lateral intrusion layer (Socolofsky et al. 2011). During the Deepwater Horizon incident, this lateral intrusion was the basis of the subsea plume of oil and dissolved hydrocarbons at about 1,000 m water depth that extended for many kilometers (Gros et al., 2017; Hazen et al., 2010; see section on The Deep Plume and Intrusion Layer). Above the intrusion layer, gas bubbles and oil droplets may continue to rise at individual terminal rise velocities and advect downstream with the local currents (Gros et al., 2017; Dissanayake et al., 2018). Eventually, oil and gas either fully dissolve, impact the seafloor of the continental slope, or reach the ocean surface (Lindo-Atichati et al., 2014). Surfacing volatile compounds evaporate into the atmosphere (DeGouw et al., 2011; Le Hénaff et al., 2012; Gros et al., 2017), whereas residual oil creates surface slicks and interacts with surface mixing processes (see section on Small-Scale Near-Surface Circulation Processes).
The near-field dynamics encompass a lateral region of up to a few kilometers’ distance from the release, within which the vertical plume and potential intrusion layer formation are contained, and larger gas bubbles and oil droplets rise to the sea surface. Outside the near-field region, spatial and temporal variability of ocean currents becomes important (as discussed in sections below on Coastal to Offshore Linkage, Circulation, and Transport and The Deep Plume and Intrusion Layer).
Two main classes of models have been applied to the evolution of the near-field oil and gas plume (Socolofsky et al., 2016; Boufadel et al., 2020). Computational fluid dynamics (CFD) models based on the LES approach were developed to simulate the complex, unsteady interaction of the oil and gas with the surrounding seawater explicitly and in detail. These models treat the oil droplets and gas bubbles in two main ways. Oil droplets and gas bubbles may be treated as a continuous distribution, allowing for larger-scale simulations (Yang et al., 2016; Fabregat et al. 2016a,b). Alternatively, individual bubbles or droplets can be tracked, allowing finer details to be revealed, but at limited scale (Fraga et al., 2016). CFD models also show the important influence of Earth’s rotation on near-field plume dynamics that affect both entrainment rates and detrainment levels.
Integral plume models, which model plume dynamics as an entity, use a cross-sectionally averaged velocity profile and solve for the variation of the flow rate, trajectory, plume width, and other plume parameters through a set of conservation equations (Johansen, 2003; Zheng et al., 2003; Dissanayake et al., 2018). These models are much less computationally expensive; hence, they lend themselves to rapid, full-scale modeling, so that predictions can be available in a matter of hours for making rapid forecasts (Barker et al., 2020). These models were extended recently to handle arbitrary bubble and droplet size distributions and to track the real-fluid equations of state and the dissolution of individual components of the oil and gas mixtures (Gros et al., 2016, 2017, 2018; Dissanayake et al., 2018). One example is the Texas A&M Oilspill Calculator (TAMOC), an open-source model available from Github (Dissanayake et al., 2018). Because these models are designed to handle the real-world scale of a blowout, Lagrangian3 particle-tracking models for the far field (from a few to many kilometers away) were coupled offline to such integral models to simulate the Deepwater Horizon spill (North et al., 2011, 2015; Paris et al., 2012; Lindo-Atichati et al., 2016; French-McCay et al., 2018; Perlin et al., 2020), as well as dynamically coupled to one another (Vaz et al., 2019). These models can track both the liquid oil droplets and the passive tracers associated with dissolved hydrocarbon in both the near-field plume and the subsea lateral intrusion (Bracco et al., 2020).
Several laboratory, tank, and field experiments have been conducted in the last 10 years and used to test and validate both CFD and integral-type models of the near field. These studies include turbulence in bubble plumes (Lai and Socolofsky 2019; B. Wang et al., 2019), the dynamics of buoyant oil jets with and without dispersant injection (Murphy et al. 2016), and field experiments to track the evolution of gas bubbles associated with natural seeps (B. Wang et al., 2016, 2020).
The most important processes occurring in the near-field plume (see, e.g., Gros et al., 2016, 2017; Dissanayake et al., 2018) include (1) partitioning of the released fluids into separate gas and liquid petroleum phases, each containing a complex mixture of the released hydrocarbons; (2) primary breakup of the gas and liquid into bubbles and droplets; (3) dissolution of many lighter compounds from the gas- and liquid-phase particles; (4) the dynamical interaction of the buoyant plume with surrounding waters; (5) rapid vertical transport of the coherent plume into the intrusion layer (if present) or to the surface (in the case of shallower releases); (6) formation of intrusions that contain most of the dissolved hydrocarbons from the plume and some of the smaller oil droplets; and finally, (7) release of remaining gas bubbles and oil droplets from the intrusion layer. Near-field models aim to accurately predict phase separation, dissolution, and intrusion formation at scales up to a few kilometers (see also the section on The Deep Plume and Intrusion Layer). Intermediate and mesoscale (from a few up to a few hundred kilometers) and far-field (larger-scale) models need to be coupled within a region following intrusion formation. Because a large volume of hydrocarbons may enter such intrusions, far-field models need to track them, along with the droplets and the dissolved oil within and around them (e.g., Vaz et al., 2019). With respect to issues requiring further research, many questions remain about oil droplet size distribution (Faillettaz et al., 2021), as discussed above in the section on Microscale Physical (and Biophysical) Processes. Improvements in the time-varying estimation of initial droplet size distribution as live oil rises from the buoyant plume into the far-field water column will likely result in the next breakthrough. These estimates should be based on our understanding of multiphase and pressure-drop processes on the gas portion of the gas-saturated droplets (Griffiths, 2012; Socolosky et al., 2016; Vaz et al., 2020; Malone et al., 2020; Faillettaz et al., 2021), their evolution through partitioning and degassing during oil ascent in the water column (Gros et al., 2016; Jaggi et al., 2017, 2020; Pesch et al., 2018) and biodegradation (Joye et al., 2016b), and on the acquired knowledge of the effect of subsea dispersant injection (Paris et al., 2012, 2018; French-McCay et al., 2019).
3 In a Lagrangian approach, observations and estimates are made at points that travel along with the flow.
Small-Scale, Near-Surface Circulation Processes
Predicting the distribution of oil, buoyant plastics, flotsam, and marine organisms near the ocean surface remains a fundamental problem of practical importance. Work on this topic during GoMRI is summarized here, with an emphasis on the accumulation of floating material into highly concentrated streaks on horizontal scales from a few meters to tens of kilometers. A more detailed review of this topic is available in D’Asaro et al. (2020).
Prior to the GoMRI period, two new paradigms for surface distributions had emerged: one for the larger scales, emphasizing the importance of submesoscale (hundreds of meters to tens of kilometers) frontal dynamics, and the other for the smaller scales, emphasizing the importance of wind-surface-wave-driven Langmuir turbulence,4 with a broad transition between the two occurring near 100 m. The small-scale motions are non-geostrophic, they are fully three-dimensional (especially at the smallest scales), and they involve substantial vertical motions that are largely confined to the near-surface mixed layer. Such motions cause both convergence and divergence of water at the surface, leading to the concentration (and sometimes subduction) of any floating materials. Pollutants, including oil, together with nutrients, Sargassum algae, larvae, and low-salinity waters of river origin therefore tend to accumulate along submesoscale fronts that are characterized by strong lateral convergence (Huntley et al., 2015; Choi et al., 2017). Such fronts may be river-
induced and thus represent a conduit that links the coastal to the open ocean, and they are key to both alongshore and cross-shore transport (Androulidakis et al., 2018) and to connectivity among coastal areas distant from one another. The importance of submesoscale motion in winter was accentuated away from the Mississippi River outflow, with perhaps the primary unanticipated result near the outflow being the remarkable and controlling influence of the associated freshwater anomalies and their expression at the submesoscale, quite likely independent of season, though dependent on river outflow rate.
In the open ocean, the Loop Current and its detached eddies (with diameters of 100–200 km) and submesoscale circulations all interact and contribute to the spreading of the riverine discharge offshore. In the northern Gulf of Mexico, submesoscale circulations are prevalent at the ocean surface not only in winter, as found in other basins, but also in summer, due to the amplification of the frontogenetic tendency associated with the abundant low-salinity water discharged by the Mississippi River system (Luo et al., 2016; Barkan et al., 2017).
Rapid progress in studying these processes has resulted from the combination of high-resolution numerical modeling tools, mostly developed before GoMRI, and new observational techniques developed during GoMRI (Mensa et al., 2018). Massive deployments of inexpensive and biodegradable satellite-tracked surface drifters combined with aerial tracking of oil surrogates (drift cards) enabled simultaneous observations of surface ocean velocities and dispersion over scales of 10 m to tens of kilometers. Surface current maps produced by ship-mounted radar and aerial optical remote sensing systems, combined with traditional oceanographic tools, enabled a set of coordinated measurement programs that supported and expanded these new paradigms (Özgökmen et al., 2018; see also sections below on Offshore Circulation and Transport and Coastal to Offshore Linkage, Circulation, and Transport).
Submesoscale fronts caused floating material to both accumulate at fronts and disperse as they evolved, leading to higher localized concentrations in some places, but nevertheless increased overall larger-scale dispersion (D’Asaro et al., 2018). Analyses of both model results and observational data confirmed the distinct submesoscale dynamics of this process and the complexity and variability of the resulting surface concentration fields. Existing analytical and modeling tools could be developed into predictive models for submesoscale processes on a statistical basis, but prediction of individual submesoscale features will likely always remain limited by the data that can be obtained and the transient nature of the processes involved. Away from fronts, measured rates of accumulation of material in and beneath surface windrows was found to be consistent with Langmuir turbulence, but highly dependent on the rise rate of the material and thus, for oil, on the droplet size (Chen et al., 2016b). Models of this process were developed and tested and could be further developed into predictive tools (Sullivan and McWilliams, 2018). Both the submesoscale and Langmuir processes are sensitive to coupling with wind and surface waves as well as air-sea interaction processes, further complicating forecasting of the submesoscale distributions of floating materials. In addition, competition between Langmuir turbulence and submesoscale dynamics has been found to be quite important, if somewhat intermittent in space and time. Finally, and importantly, boundary layer turbulence due to an external source, such as wind or convection, can couple to the submesoscale dynamics via a turbulent thermal wind effect5 on the cross-front balance between the pressure gradient and the Coriolis force. Here, both the turbulent intensities and the submesoscale frontogenesis can be affected. The interactions discussed here provide important directions for future research.
4 Langmuir turbulence is the near-surface three-dimensional turbulent motion associated with Langmuir circulation cells. They are long, shallow overturning cells that form when surface currents interact with wind-driven waves.
5 When a balanced front is disturbed by turbulent mixing, a secondary circulation with flow in the cross-front and vertical directions develops. The horizontal convergence associated with the secondary circulation intensifies development of the front (see, e.g., Crowe and Taylor, 2018)
Offshore Circulation and Transport
When hydrocarbons from a deep oil spill reach the surface, they are strongly influenced by air-sea forcing, including surface dispersion by ocean currents, mixed layer dynamics, wind, and waves, all of which vary greatly over time and with weather conditions (Judt et al., 2016). The impacts of processes operating over a very wide range of spatial and temporal scales must therefore be taken into account when responding to an oil spill. Advection of the oil by large-scale flows (tens to hundreds of kilometers, for example, the Loop Current and associated eddies) provides a first-order description of the oil’s trajectory, but small-scale (100 m to 10 km) processes critically impact transport and mixing in the upper ocean. In particular, Stokes drift6 from surface waves and Ekman transport from wind stress combine to form the near-surface currents that advect the oil. The depth of such currents is controlled by boundary layer turbulence, including Langmuir circulations, that are driven by air-sea fluxes and surface waves. These processes create complex distributions of material and produce very different patterns of the oil spread than would be obtained considering only the larger-scale flows.
During GoMRI, the Gulf of Mexico’s near-surface circulation was investigated by deploying thousands of biodegradable Lagrangian drifters, notably in the Grand LAgrangian Deployment (GLAD) and the LAgrangian Submesoscale ExpeRiment (LASER) (Novelli et al., 2017) of the Consortium for Advanced Research on Transport of Hydrocarbon in the Environment (CARTHE). Lagrangian deployments are naturally suited for studies of the tracer transport problem because they do not suffer from the sampling and averaging errors of fixed and ship-based measurements, and they continue to sample the velocity field long after the cruise period ends, even under adverse conditions. Cumulative trajectories from CARTHE’s sampling of the Gulf of Mexico are shown in Figure 3. After being released near the DWH site, drifters dispersed across almost the entire basin (with the exception of continental shelves) in three to six months. A comparison with the oil concentration from the DWH event shows that the GLAD (summer) and undrogued LASER (winter) drifters have a very similar spatial distribution to that of oil from the DWH spill, while drogued drifters from LASER tend to follow a mesoscale filament southward all the way to the Mexican shelf break (Haza et al., 2018, 2019). This led to the conclusion that upper-ocean vertical shear and seasonality play important roles in transport patterns. It is important to keep in mind that, over the long term (weeks, months), drifters differ from oil in many ways, in that they do not evaporate or undergo other complex transformations.
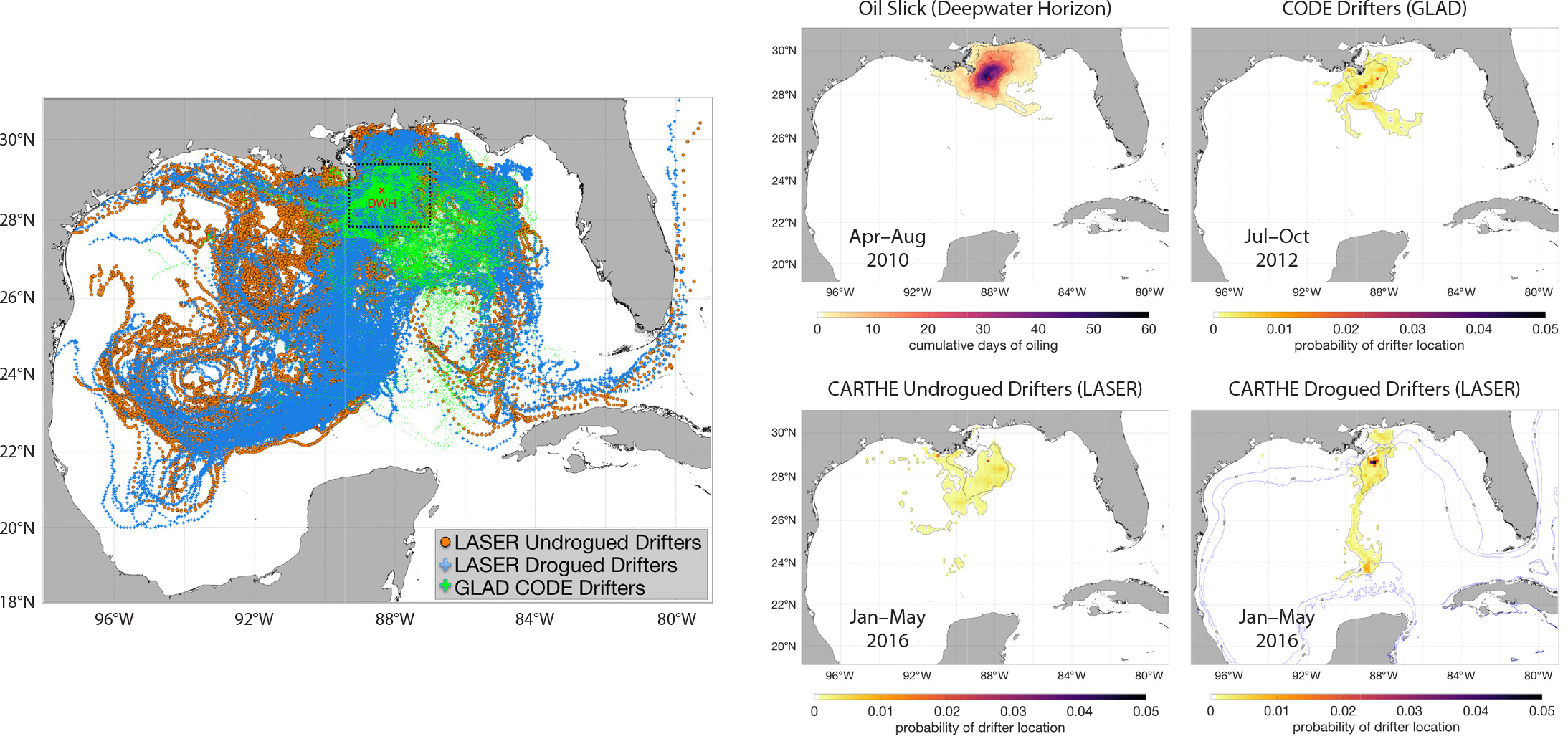
Figure 3. (left panel) Cumulative spatial span of all Consortium for Advanced Research on Transport of Hydrocarbon in the Environment (CARTHE) drifters deployed in the northern Gulf of Mexico. (right panels) Oil coverage from the DWH event compared to drifter density from the Grand LAgrangian Deployment (GLAD) and LAgrangian Submesoscale ExpeRiment (LASER), with drogued and undrogued drifters shown separately. From Novelli et al. (2020) > High res figure
|
Coupled atmosphere-wave-ocean models can be used in conjunction with the drifter measurements. Figure 4 displays model-simulated drifter transport pathways for the winters of 2015, 2016, and 2017 from the Unified Wave Interface-Coupled Model (UWIN-CM; Chen et al., 2013; Chen and Curcic, 2016) with both atmospheric and oceanic components fully coupled to the surface wave model. Thus, it allows for an accurate exploration of wind-wave-current coupling and the influence of Stokes drift on surface material transport. The transport patterns are modulated to a large degree by the structure of the Loop Current, whose northern intrusion varied greatly during 2015–2017. These three years correspond to the 2015–2016 El Niño and the subsequent weak La Niña in 2017. The area of the spill and the transport of simulated drifters out of the Gulf through the Florida Straits both depend on the structure of the Loop Current, while coastal beaching remains more or less the same across these three years and must therefore depend on smaller-scale features of the circulation. The UWIN-CM simulations and the LASER drifter data for 2016 show reasonable agreement. Wind-induced surface waves enlarge the area of spilled oil in general (denoted as Lagrangian vs. Eulerian in Figure 4h,i), which can affect how oil and drifters interact with the Loop Current. The simulated Lagrangian drifters spread faster over a larger area than the simulated Eulerian drifters, which remain more concentrated near the Loop Current and may be transported out of the Gulf. Overall, Figure 4 provides a rather striking picture of multiscale air-sea interaction, especially the interannual variability of surface transport patterns in the entire Gulf of Mexico.
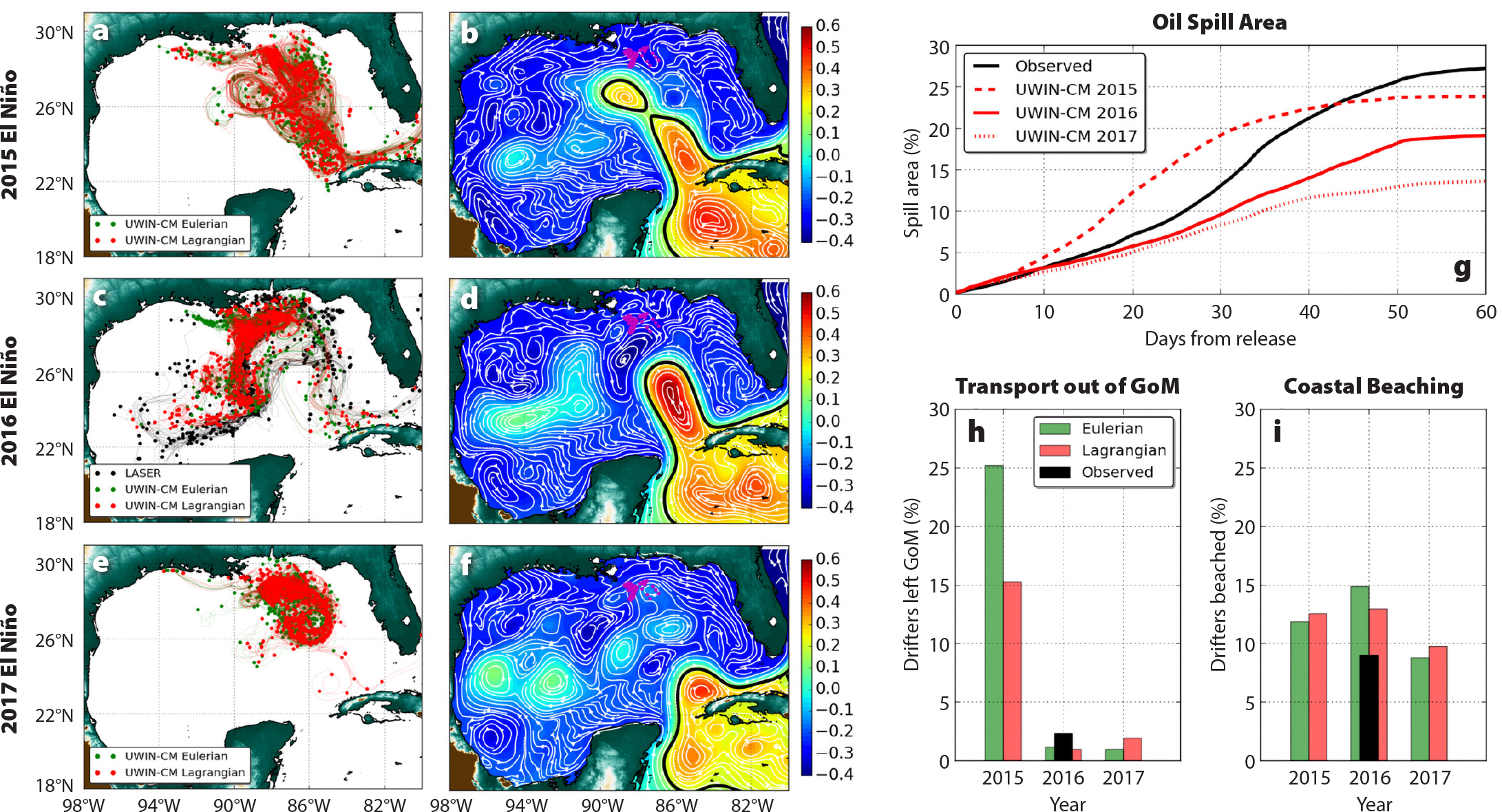
Figure 4. (LEFT PANELS) Unified Wave Interface-Coupled Model (UWIN-CM) simulations of Gulf of Mexico (GoM) circulation. Panels (a), (c), and (e) show simulated Eulerian (green) and Lagrangian trajectories (red), and panels (b), (d), and (f) illustrate average sea surface height (SSH, in colors) and surface circulation (streamlines, in white) between January 21 and March 29 in 2015, 2016, and 2017. Panel (c) also shows observed LASER trajectories (black). Initial drifter locations are indicated with magenta dots near the DWH site. The Loop Current is shown by the 17 cm SSH contour (thick black line). (RIGHT PANELS) Simulated and observed characters of the oil spill: (g) evolution of oil spill area, (h) transport out of the Gulf, and (i) coastal beaching for 2015–2017 (data supplied by author Chen). > High res figure
|
Near-field, short-term dispersion is, however, more important than long-term transport for guiding a spill response effort. To this end, local and real-time assimilation of data to initialize and constrain the models, using adequate data sources and good assimilation schemes, is critical for a good forecast. For example, the upper left panel of Figure 5 shows Lagrangian coherent structures (LCSs)7 computed from the Navy Coastal Ocean Model (NCOM) during the GLAD expedition. There is a significant mismatch with a concurrent chlorophyll image collected by the Moderate Resolution Imaging Spectroradiometer (MODIS). In fact, Jacobs et al. (2014) found that LCSs computed from satellite-altimeter-derived geostrophic velocity directly, without assimilating into NCOM, initially were in better agreement with this chlorophyll image. This implied the need for modification of procedures followed during data assimilation into NCOM when there was a scarcity of altimeter tracks over the Gulf of Mexico. After the procedures were modified, much better agreement was attained (lower left panel of Figure 5). Assimilation of data from the large numbers of drifters further improved model performance. By developing appropriate Lagrangian data assimilation algorithms, Carrier et al. (2014) and Muscarella et al. (2015) found that the assimilation of even a small number of (GLAD) drifter trajectories improved NCOM realism (right panels of Figure 5). In particular, for oil spills, drifters can be deployed repeatedly right on top of the spill to track it during nighttime and bad weather, as opposed to hoping that satellite tracks will coincide with the spill’s location. However, because results at scales that are not constrained by data become chaotic and do not have useful forecasting value, high-resolution modeling requires high-resolution data. By themselves, however, high-resolution models may still provide insight into the potential ensemble of possible flow characteristics, and on the importance of the various physical processes at play.
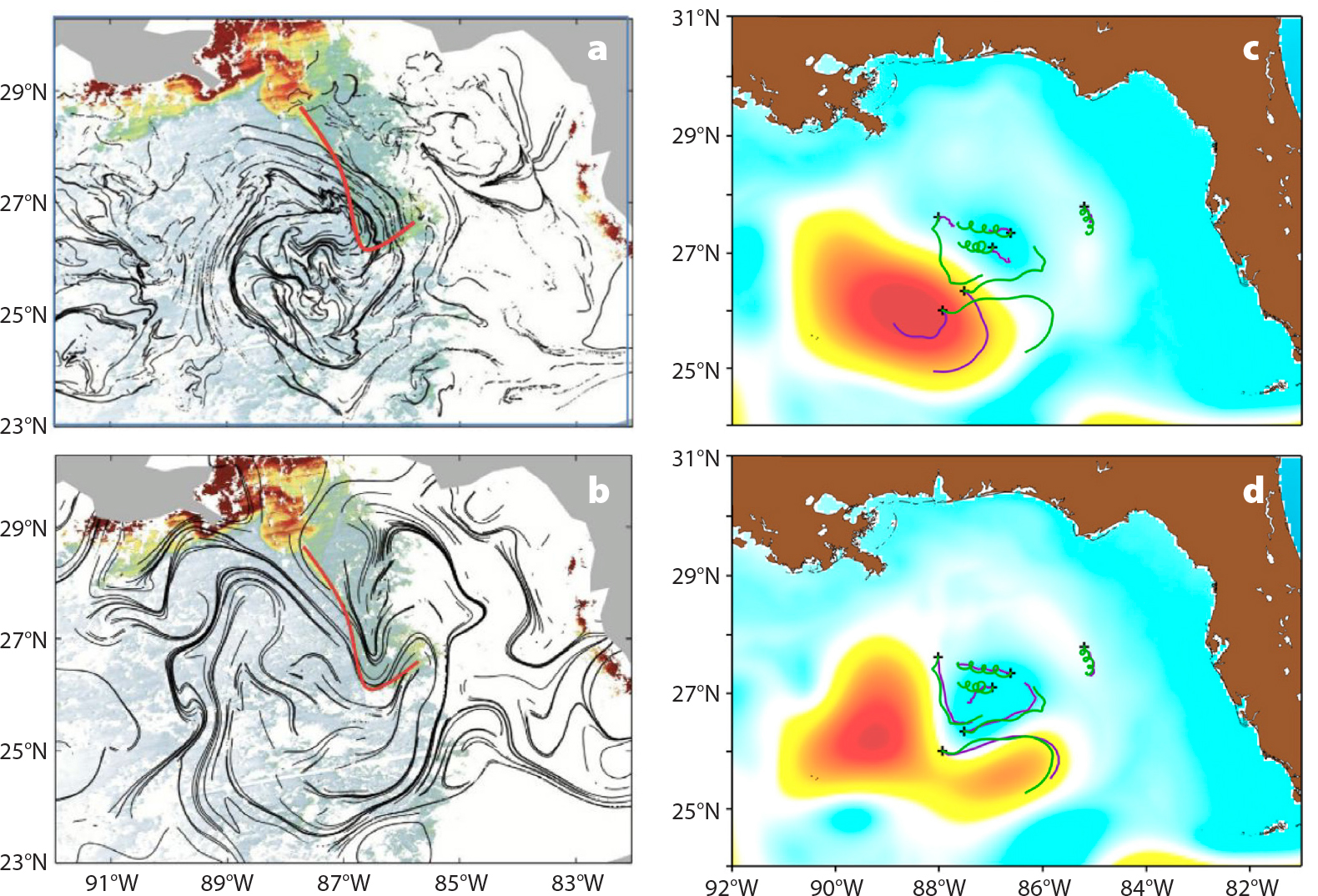
Figure 5. Improvement of model predictions using data assimilation. (left panels) Navy Coastal Ocean Model (NCOM) simulation of particle tracks with superimposed sea color images, both without (a) and with (b) assimilation of altimeter data. Note the improved alignment of the flow field with the long filamentary “intrusion.” From Jacobs et al. (2014) (right panels) Further improvement of NCOM simulations, both without (c) and with (d) assimilation of GLAD drifter data. Note the improved alignment of fronts with drifter tracks. From Muscarella et al. (2015). > High res figure
|
It should be noted that not all of the oil from the Deepwater Horizon blowout reached the surface. A substantial percentage of the oil was entrained into deep plumes and biodegraded therein. The behavior of such plumes is not easily predictable, because of lack of knowledge of the deep circulation and how it is modified by diapycnal mixing. This issue is discussed in detail in section below on The Deep Plume and Intrusion Layer.
6 Stokes drift is the systematic net motion of material particles in a surface wave field caused by the difference between the Lagrangian and Eulerian average velocities, effectively the difference in particle velocities between the top and the bottom of their approximately circular trajectories.
7 Lagrangian coherent structures are systematic patterns of circulation within a turbulent flow field that lead to the formation of coherent patterns in the distribution of tracer materials (e.g., blobs and streaks).
Coastal to Offshore Linkage, Circulation, and Transport
Under the auspices of GoMRI, much has been learned about transport processes in the Gulf of Mexico along the very important land-ocean continuum, from the coast, estuaries, wetlands and shelves to the deep ocean interior. An in-depth synthesis of this research will be available in a forthcoming paper by author Justić and colleagues. Advances have been particularly outstanding with respect to the tools and methodologies available for collecting, analyzing, and synthesizing data and for simulating coastal to offshore linkages and transport. Taken together, these improvements enable better short- and long-term forecasting capabilities as well as better-informed decisions for coastal resource and ecosystem management. Below, we summarize major progress in a geographical progression, moving from the land-ocean boundary to the open ocean.
Observations, laboratory experiments, and data analyses have all contributed to a better understanding of estuarine and coastal hydrodynamics and associated transport of freshwater, sediments, nutrients, and pollutants (Zheng and Weisberg, 2010; Beland et al., 2017; Valentine and Mariotti, 2019). The unstructured grid models that are now available are better equipped to simulate the complex coastal geometries of marshes, wetlands, and estuaries, and their multiscale time variabilities (Justić and Wang, 2014; White et al., 2019c). There are also improvements in modeling of river-estuarine coupling, and there is widespread assimilation of operational meteorological or reanalysis products. For example, we have come to realize that river diversions influence estuary dynamics within 20–25 km from the diversion, but they may not significantly impact the transport of oil slicks further offshore (H. Huang et al., 2011). However, large-scale sediment diversions have the potential to influence estuarine flushing further than 60 km from the diversion sites, albeit with significant ecological trade-offs associated with estuarine freshening (Cui, 2018). The emerging consensus on the use of river diversions in the case of DWH is that they were ineffective in achieving their primary objective to push the oil away from coastal wetlands and rather resulted in unanticipated deleterious ecological impacts (Westerholm et al., 2021, in this issue). This was due to the complex interaction of Mississippi River plume waters with the oil that was moving onshore (Kourafalou and Androulidakis, 2013), interaction that was dependent on the offshore currents over the shelf (dominated by wind and river discharge variability) and on the open sea (dominated by the Loop Current and associated eddies).
In the past 10 years, we have come to appreciate the importance of both horizontal and vertical resolution in coastal and regional models. High vertical resolution is required to achieve good representation of the Ekman layers at the ocean surface and near the seafloor (within which currents are diminished and rotated by the joint effects of viscous drag and the Coriolis force; Weisberg et al., 2014) and to properly capture diapycnal8 mixing in both coastal areas and in the open ocean (Bracco et al., 2018). Furthermore, at the land-ocean interface, especially in the presence of barrier islands and beaches, representation of flooding and drying is important and should be directly included in the vertical coordinate system (Zheng and Weisberg, 2012; Weisberg et al., 2014). High-resolution coastal and regional models should pay close attention to the representation of inlets and islands (Androulidakis et al., 2019, 2020) and the effects of wind-wave-current coupling on transport due to Stokes drift (Curcic et al., 2016), whose influence varies depending on water depth. Stokes drift did indeed contribute to the deposition of oil on beaches following the DWH spill (Le Hénaff et al., 2012).
Finally, major refinements of transport modeling tools have improved our ability to predict ecosystem connectivity (the interrelationships within and between species in different places) and oil transport. On the ecosystem side, we have made much progress in understanding and predicting movement of adult and juvenile fish, coral larvae, and other taxa (Cardona et al., 2016; Ainsworth et al., 2018; Martin et al., 2020; Paris et al., 2020) and in estimating connectivity among coastal habitats, shelves, and estuarine wetlands of the Gulf of Mexico. Regarding the transport of oil, the initial surface trajectory models used as part of the rapid response to the DWH spill have been augmented by simulations that include oil droplet size variations and subsea chemical dispersant applications, using both Eulerian9 and Lagrangian approaches. Through modeling and observational efforts, we have better quantified the important role that Stokes drift played in modifying the dispersion of the oil toward both the coastline and open waters. We have also realized that river diversions have limited effectiveness in keeping the oil away from the estuaries (H. Huang et al., 2011), that the Mississippi River plume exerts buoyancy-driven effects on the oil that may vary significantly in space (Dagestad et al., 2018; Hole et al., 2019), and that there is an elevated risk of remobilization of oil stored in shallow sediments during energetic storms (Zengel et al., 2015).
Challenges remain in both the observational and the modeling realms. They pertain to the high computational costs associated with current algorithms for simulating wetlands, estuaries, river-estuary coupling, and shelf exchanges at the high spatial resolution needed. The mechanisms controlling wave-current interactions in river deltas and the relative importance of atmospheric fluxes, riverine fluxes, and ocean circulations also remain uncertain, especially in rough weather conditions. When investigating transport across and along coastal areas, better coordination of observational and modeling efforts should be strongly encouraged; the uncertainties in atmospheric forcing and boundary conditions (wind stress and heat flux) should be quantified and, if possible, reduced; and simulations of circulations around barrier islands and beaches should have longer time horizons. A multiscale approach, using multiple models at different scales simultaneously through nesting techniques, is recommended. Such an approach should be augmented by an in-depth exploration of mesoscale-submesoscale linkages and by an investigation of model dependency on vertical discretization. Riverine forcing should be better monitored and accurately modeled not only to improve forecasting and response planning in the event of another spill but also to better monitor and manage the Gulf marine ecosystem. In this regard, more observations are needed to constrain how species behavior contributes to coastal-open ocean ecological linkages. Finally, further model intercomparison studies using different hydrodynamic and oil (particle-tracking) models should be prioritized in order to improve model formulations for oil-tracking purposes.
In summary, much progress in understanding transport processes has been achieved in the past 10 years. To sustain such growth and amplify its benefits, we recommend the development of a suite of appropriately scaled ocean circulation and wave models that are capable of strategically zooming into the key components of the land-ocean continuum, and that are supported by observing arrays designed to target process understanding, data assimilation, and model validation.
8 Mixing in the deep ocean occurs predominantly along isopycnals (the approximately horizontal surfaces of constant density). A much smaller but nevertheless extremely important amount of mixing occurs across or between density surfaces, known as diapycnal mixing.
9 In an Eulerian approach, observations and estimates are made at fixed points in space, whereas in a Lagrangian approach they are made at points that travel along with the flow.
The Deep Plume and Intrusion Layer
As mentioned in the section on Offshore Circulation and Transport, not all the oil from the DWH blowout reached the surface. A significant proportion (up to 50%) was entrained into deep subsurface plumes or intrusion layers that traveled laterally at approximately 1,200 m depth along the continental slope and was biodegraded therein. The largest and best sampled of these was discovered through in situ measurements in August 2010 (Camilli et al., 2010; Diercks et al., 2010; Kessler et al., 2011). Since its discovery, the scientific community has made significant progress in understanding and modeling the transport, evolution, and fate of the hydrocarbons trapped in the plume (Gros et al. 2017; also reviewed in Bracco et al., 2020). However, a major challenge for data assimilative ocean models that seek to predict the circulation of the Gulf of Mexico below 1,000 m depth remains due to the absence of any continuous monitoring systems that extend below the ocean’s surface (Cardona and Bracco, 2016).
The deep tracer release experiment by the GoMRI-funded Gulf of Mexico Integrated Spill Response (GISR) Consortium was designed to address the limited knowledge of key physical variables below the mixed layer by providing an estimate of interior diapycnal mixing. In August 2012, Ledwell and collaborators from the GISR Consortium injected a streak of trifluoromethyl sulfur pentafluoride (CF3SF5) at about 1,100 m depth near the DWH site to quantify its dispersion (Ledwell et al., 2016). The tracer was sampled one week, four months, and finally one year after its release. A turbulent diapycnal eddy diffusivity greater than 4 × 10–4 m2 s–1 was estimated for the boundary region (close to the seafloor of the continental slope), which is very high relative to typical values for the deep ocean interior (<10–5 m2 s–1), but lower values were found for the interior of the plume. The main conclusion from this exercise was that diapycnal mixing at mid-depth in the northern Gulf of Mexico is much greater near the continental slope than in the interior and that homogenization of a tracer released near the continental slope proceeds much faster than in the open ocean (Polzin et al., 2014). These effects are not yet included in operational models.
Bracco et al. (2016) examined the generation mechanisms and mixing implications of mesoscale and submesoscale circulations near the ocean floor. They showed that the diapycnal mixing coefficient increased near to the continental slope compared to the interior, as observed in the tracer data, and also to the west of the Mississippi Fan, where submesoscale processes were more abundant compared to De Soto Canyon. Later, the contribution of horizontal and vertical model resolutions to the representation of diapycnal diffusivities and the advection of a passive tracer was further explored (Bracco et al., 2018, 2019). Results suggested that a deep spill that occurred to the west of the Mississippi Fan would impact the continental slope far more extensively than did the DWH spill by mixing the hydrocarbons more rapidly and distributing them over a larger area in a few months (Rogener et al., 2018).
In terms of hydrocarbon modeling, major improvements made include better estimations of the initial droplet size (Bandara and Yapa, 2011; Paris et al., 2012; Johansen et al., 2013; Zhao et al., 2014a,b, 2016; Aman et al., 2015; C. Li et al., 2017; Malone et al., 2018; Pesch et al., 2020a) and more accurate representations of plume dynamics (Paris et al., 2012; Lindo-Atichati et al., 2016) and of the pressure-dependent process of internal degassing in two-phase droplets (Pesch et al., 2018, 2020b). Focusing here on the representation of the lateral plume evolution in the far field, state-of-the-art models simulate the advection of individual droplets by the velocity field generated in hydrodynamic models (North et al. 2011, 2015; Paris et al., 2013; Perlin et al., 2020) and the dissolution and biodegradation of gas and oil droplets as functions of the local environmental and biogeochemical conditions (Chen et al., 2016a; Joye et al., 2016a,b). It is now also possible to realistically simulate droplet aggregation with both organic and inorganic matter and the subsequent settlement of the heavier aggregates on the seafloor (Khelifa et al., 2008; Zhao et al., 2016; Daly et al., 2016, 2020; Dissanayake et al., 2018; Vaz et al., 2020), and also to allow for the effect of subsea dispersant injection (Paris et al., 2012, 2018; French-McCay et al., 2019).
With respect to issues requiring further research, many questions remain concerning the spatial and temporal evolution of flows in midwater and in the bottom boundary layer, and specifically how processes at scales of centimeters to hundreds of meters translate into large-scale transport impacts (Bracco et al., 2020). Improved theoretical understanding of ocean near-bottom dynamics is needed and could be achieved with a few years of targeted monitoring using acoustic Doppler current profilers (ADCPs) to quantify velocities and their variability, while the current generation of high-resolution, regional models could identify locations to prioritize for the observational efforts. Because detection and modeling of subsurface oil is difficult, advances will also emerge from the use of ensemble probabilistic modeling (Perlin et al., 2020) for guiding underwater remotely operated vehicles. Finally, intercomparison of modeling strategies (e.g., Socolofsky et al., 2015) should be prioritized to improve the predictions of deep plumes and the response trade-offs for any future deep-sea spills (Murawski et al., 2019).
Conclusions and Key Findings
While the physical processes described above constitute dominant influences on the spatial distribution of materials including oil, a major discovery has also been the importance of the marine oil snow sedimentation and flocculent accumulation (MOSSFA) process, as described by Farrington et al. (2021) and Quigg et al. (2021), both in this issue. Here, physical, chemical, and biological processes interact to produce negatively buoyant particles that sink to the seafloor, countering the general tendency of oil to rise toward the surface. Further notable conclusions are:
- Major advances in understanding of pressure-induced processes on gas-saturated oil and refinements of transport modeling tools have improved our ability to predict both ecosystem connectivity and oil transport.
- CFD models have shown the importance of Earth’s rotation in near-field plume dynamics that affect both entrainment rates and detrainment levels.
- Recent model improvements include high-resolution regional coupled atmosphere-wave-ocean modeling, river-estuarine coupling, ability to resolve surface and bottom Ekman layers, consideration of Stokes drift, and the widespread adoption of operational meteorological or reanalysis products.
- There is an emerging consensus that the use of river diversions in the case of DWH was ineffective in achieving its primary objective to push the oil away from coastal wetlands and, rather, that it resulted in unanticipated deleterious ecological impacts.
- River-induced fronts play important roles in alongshore and cross-shore transport, acting both as barriers to material transport and as convergence zones that guide transport along frontal lines.
- Advection of pollutants along pathways that link coastal areas and the open ocean is influenced by both mesoscale and submesoscale circulation features.
- Better understanding of the effects of multiscale atmosphere-wave-ocean coupling on ocean transport, on hourly to interannual timescales, can help improve prediction not only in the Gulf of Mexico but also elsewhere.
Under the auspices of GoMRI, important advances were thus made with respect to the tools and methodologies for collecting, analyzing, and synthesizing data and for simulating coastal to offshore and deep-sea linkages and transport. To sustain such progress, and amplify its benefits, we recommend developing and maintaining a regional forecasting system in order to be better prepared for any future oil spill events. It should be based on a suite of appropriately scaled ocean circulation, atmospheric, and wave models capable of strategically zooming in on the key components of the land-ocean continuum and should be supported by well-designed observing arrays that target process understanding as well as data assimilation and model validation.